Hypothesis testing helps you make decisions about a population using sample data. It involves setting a null hypothesis (no effect) and an alternative hypothesis (effect exists), then collecting data to see if there’s enough evidence to reject the null. Picking the right significance level and understanding sample data are essential. If you want to understand how this process works and avoid common mistakes, you’ll find more helpful insights below.
Key Takeaways
- Hypothesis testing verifies assumptions about a population using sample data to determine if effects are statistically significant.
- It involves formulating null and alternative hypotheses, selecting appropriate tests, and interpreting p-values.
- The process includes collecting representative data, calculating test statistics, and comparing results to critical values.
- Choosing the correct significance level (e.g., 0.05) balances the risk of false positives and negatives.
- Proper application requires understanding data types, assumptions, and avoiding common mistakes for valid conclusions.
What Is Hypothesis Testing and Why Is It Important?
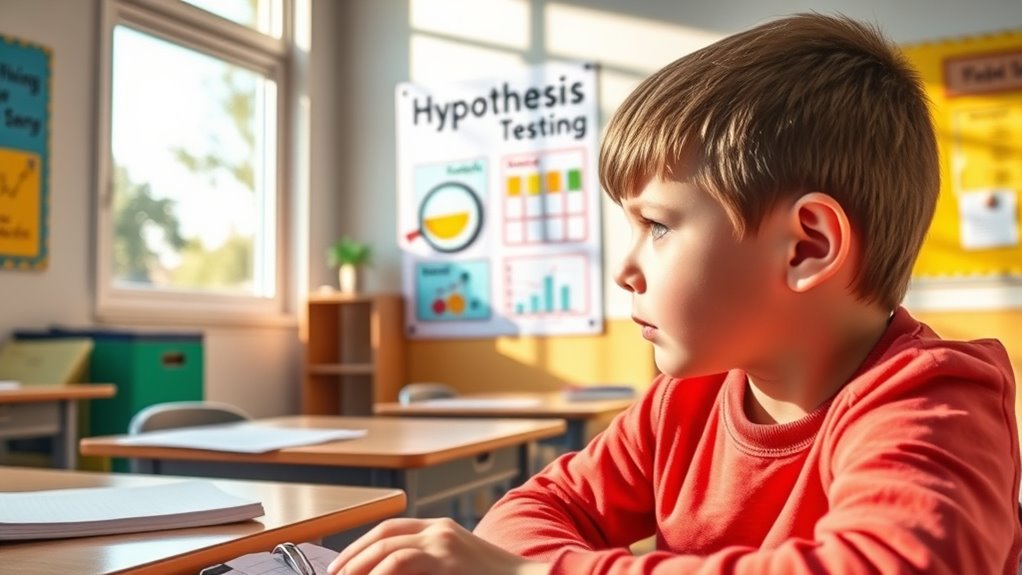
Hypothesis testing is a fundamental statistical method that helps you verify assumptions about a population based on sample data. You use it to determine whether your data provides enough evidence to reject a specific belief or hypothesis.
This process is essential because it allows you to make informed, data-driven decisions in various fields like medicine, business, and science. By setting null and alternative hypotheses, you can evaluate if observed results are due to chance or indicate a real effect. TikTok’s popularity demonstrates how viral trends can influence perceptions and behaviors, highlighting the importance of rigorous analysis in understanding social phenomena. Additionally, understanding sampling methods helps ensure that your data accurately reflects the population you’re studying.
The outcome guides your next steps—either rejecting or failing to reject the null hypothesis. Overall, hypothesis testing helps reduce errors, validate research, and advance scientific knowledge, making it a vital tool for analyzing data accurately and reliably.
The Basic Steps to Conduct a Hypothesis Test
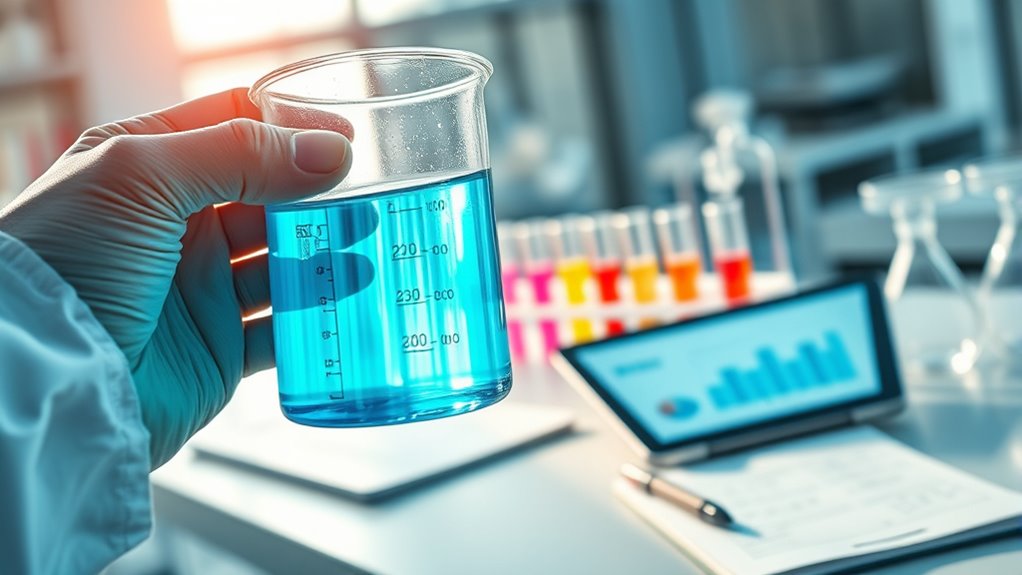
Conducting a hypothesis test involves a series of systematic steps that guide you from formulating your initial assumptions to making informed conclusions.
First, you develop your hypotheses, clearly stating the null hypothesis (no effect) and the alternative hypothesis (there is an effect).
Begin by clearly formulating your null and alternative hypotheses to guide your analysis.
Next, you collect data by selecting a representative sample from your population, ensuring the data aligns with your research question.
Then, you choose an appropriate statistical test based on your data type and distribution, such as a t-test or chi-square test.
Afterward, you calculate the test statistic and determine the p-value or critical region, considering your significance level (alpha).
Additionally, understanding how self watering plant pots work can help inform proper data collection and interpretation in related experiments.
Being familiar with industry trends can also aid in selecting the most relevant statistical methods for your analysis.
Finally, you interpret the results, deciding whether to reject or fail to reject the null hypothesis and reporting your findings accurately.
Understanding Null and Alternative Hypotheses
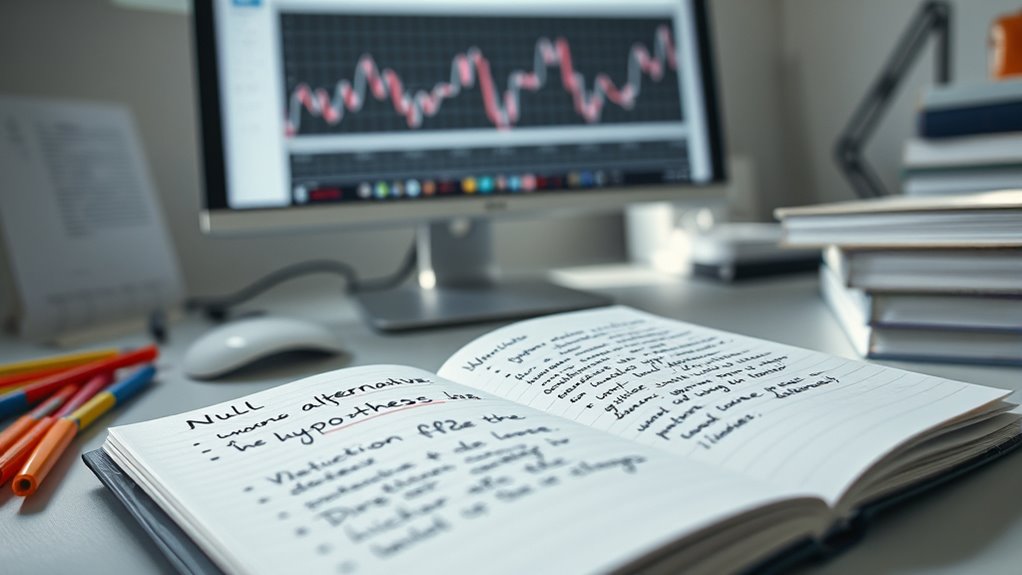
Understanding null and alternative hypotheses is essential because they form the foundation of any hypothesis test. The null hypothesis claims there’s no effect or difference in the population, like no change in test scores between two teaching methods.
The alternative hypothesis challenges this, suggesting there’s an effect or difference. Both hypotheses answer your research question by making competing claims about the population, based on sample data.
The null often states “no effect,” while the alternative indicates a “presence of effect.” You test these hypotheses statistically — using p-values, confidence intervals, and significance levels — to see which one your data supports.
How to Choose the Right Significance Level
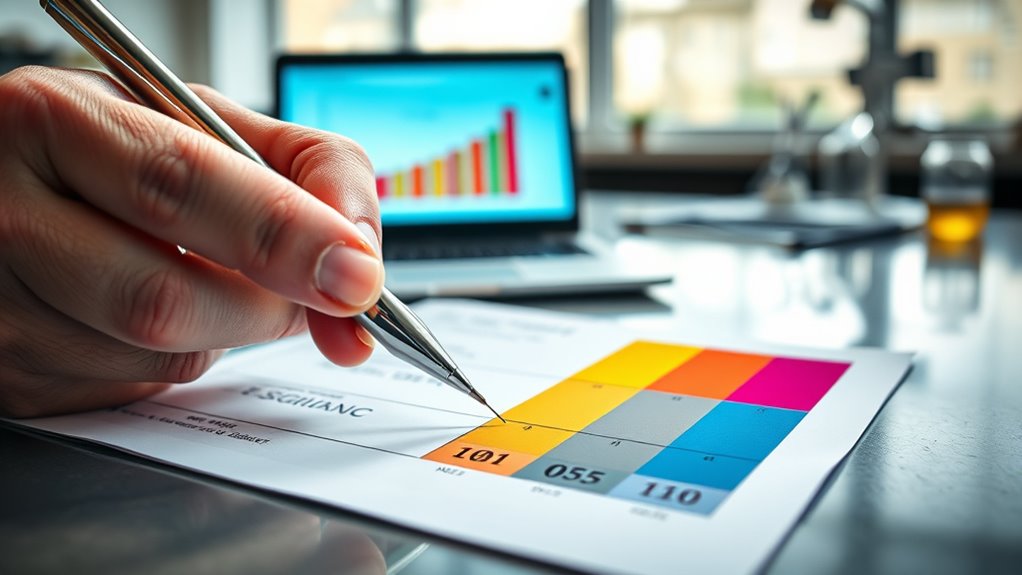
Choosing the right significance level is crucial because it directly influences how confidently you can reject the null hypothesis. Your choice depends on balancing the risk of false positives (type I errors) against the need to detect real effects. A common level is 0.05, but stricter levels like 0.01 reduce false positives, especially in critical studies such as clinical trials. Factors like sample size and expected effect size guide this decision—larger samples often allow for more stringent levels. Consider your research goals, the consequences of errors, and the field’s standards. For example, more rigorous studies might adopt lower significance levels to ensure robustness. Additionally, understanding the context of your hypothesis testing helps in selecting an appropriate significance level. Properly controlling error rates is essential to maintain the integrity of your results. Ultimately, selecting the appropriate significance level helps maintain the integrity of your conclusions and aligns with the study’s context.
Analyzing Sample Data Effectively
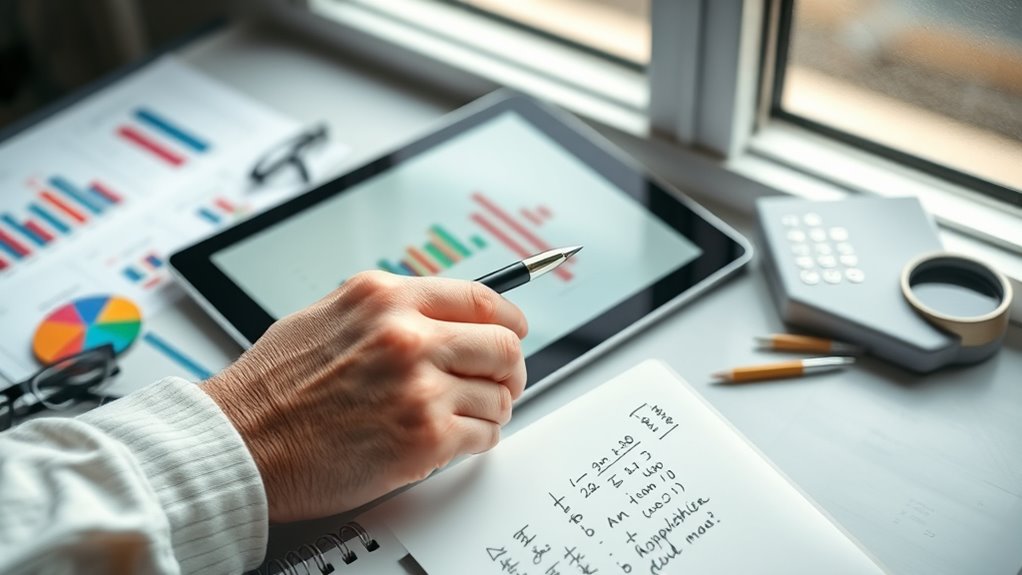
Effectively analyzing sample data is essential for drawing valid conclusions in hypothesis testing. First, ensure your sample size is appropriate to boost the test’s power and reliability. Use random sampling techniques to make sure your sample accurately represents the population, and understand key parameters like mean and standard deviation.
Recognize the data type—whether continuous, categorical, or ordinal—to select suitable tests. Before proceeding, evaluate distribution assumptions by conducting normality tests, checking for equal variances, and identifying outliers through plots or statistical methods. Sometimes, transforming data helps meet assumptions.
Accurate calculations of test statistics, like Z or T scores, depend on understanding the data and applying formulas correctly. Using software tools can improve accuracy and efficiency in your analysis. Additionally, staying informed about latest breakthroughs in machine learning tech can provide insights into advanced analytical methods and tools that enhance hypothesis testing accuracy.
Making Decisions: Critical Values and P-Values
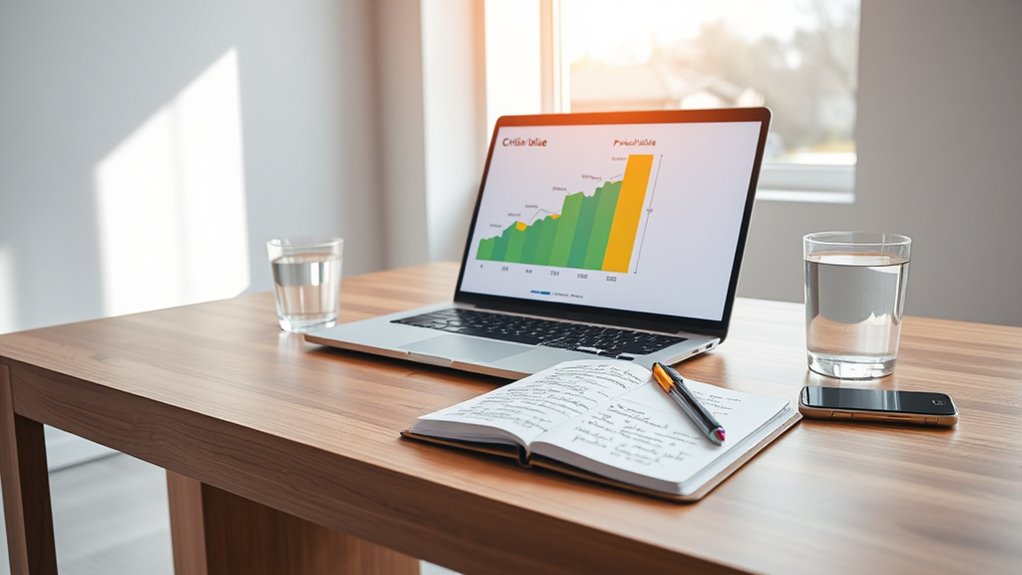
Once you’ve collected and analyzed your sample data, the next step is to decide whether to reject the null hypothesis. You do this by comparing your test statistic to a critical value or by examining the p-value. Critical values are predefined points on the distribution that mark the boundary of the rejection region, based on your significance level (α). If your test statistic falls into this region, you reject the null hypothesis. Alternatively, with the p-value method, you compare the p-value to α. If the p-value is less than α, it indicates strong evidence against the null hypothesis, leading to rejection. Both methods help you make an informed decision, but they approach the problem differently—one uses thresholds, the other focuses on probabilities. Recognizing the importance of support hours in entertainment and parks can influence how you interpret the results of hypothesis tests in social science research.
Interpreting the Results of Your Test
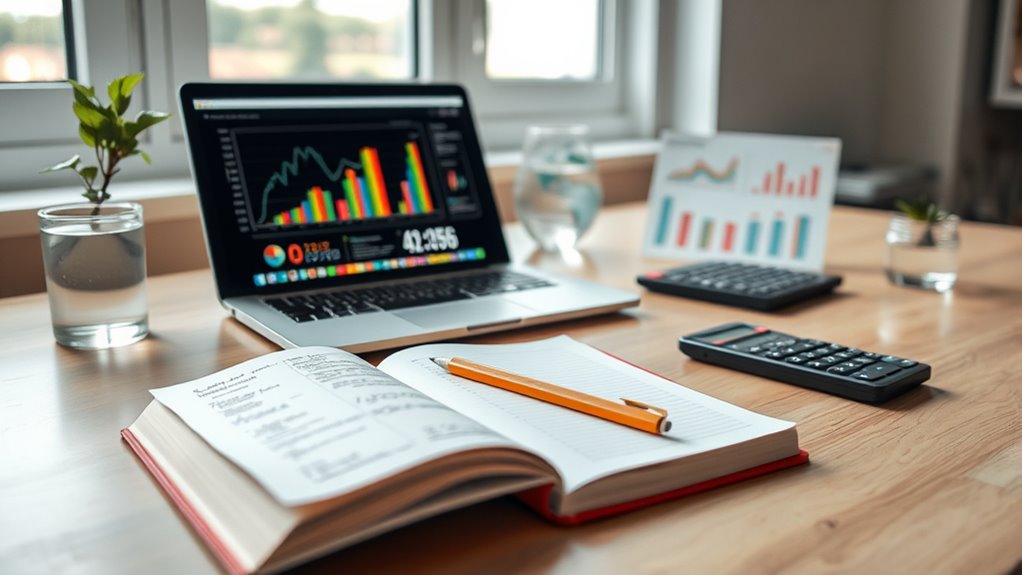
Interpreting the results of your hypothesis test is essential for understanding what the data reveals about your research question. First, check the p-value: if it’s less than or equal to your significance level (often 0.05), you reject the null hypothesis, indicating the results are statistically significant. A small p-value suggests the observed data is unlikely under the null hypothesis. Remember, failing to reject the null doesn’t prove it; it simply means there’s not enough evidence to do so. Always report the p-value clearly and interpret it within your research context. Consider practical significance alongside statistical significance, and be aware of potential errors like Type I or II. Use these insights to guide your conclusions and inform your next steps. Additionally, understanding the performance metrics associated with your analysis can help better interpret the reliability and impact of your findings. For example, utilizing crochet styles for locs can serve as an analogy for exploring different approaches to data interpretation, emphasizing the importance of diverse methods in reaching accurate conclusions.
Different Types of Hypotheses and Tests
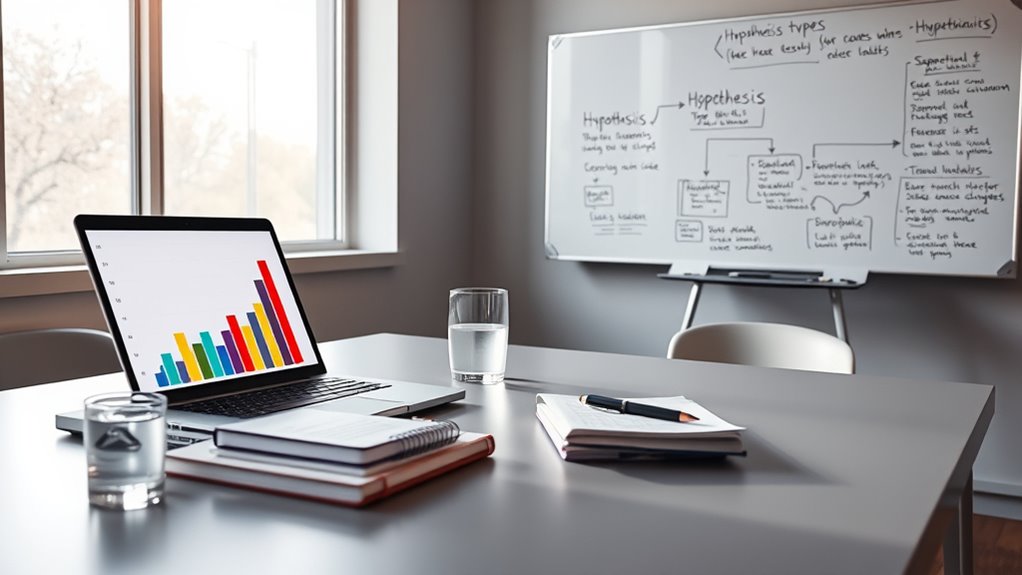
Understanding the different types of hypotheses and tests is fundamental to designing and analyzing research studies. You’ll encounter null hypotheses, which claim no effect or relationship between variables, and alternative hypotheses, suggesting an effect exists.
Hypotheses can be simple, involving one independent and dependent variable, or complex, involving multiple variables. You might also work with composite hypotheses, which specify a range of possible values. For instance, the power output of electric dirt bikes ranges from 10 to 20 horsepower, influencing their performance and categorization.
Directionality is another important aspect. Directional hypotheses predict the specific direction of a relationship, while non-directional ones do not.
Associative hypotheses suggest a correlation, whereas causal hypotheses propose cause-and-effect links. Recognizing these variations helps you choose the appropriate statistical tests and interpret results accurately, ensuring your research design aligns with your hypotheses and research goals. Personality Test concepts can also inform hypotheses by providing insights into individual differences that may influence outcomes.
Common Mistakes to Avoid in Hypothesis Testing
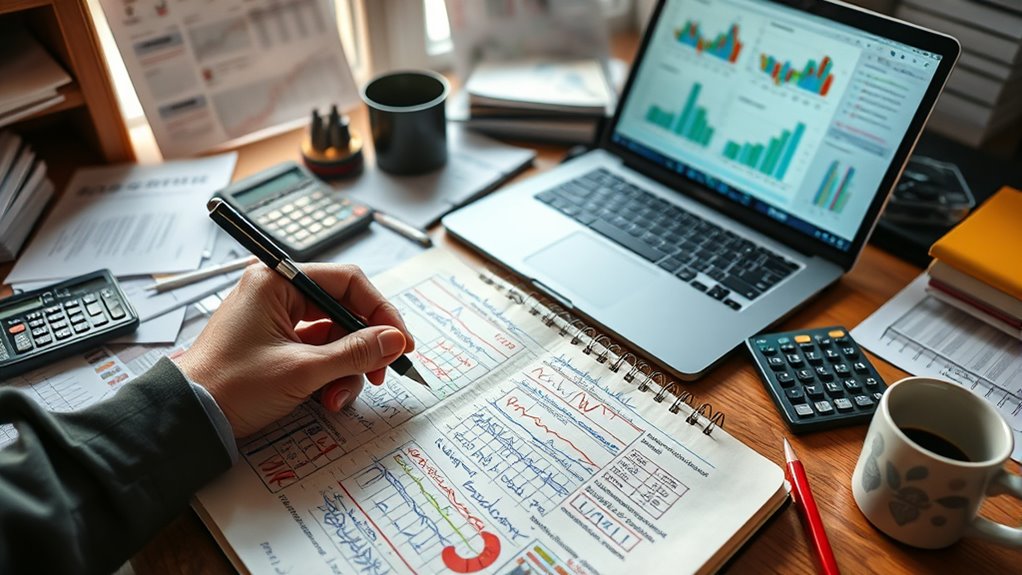
Mistakes in hypothesis testing can undermine the validity of your research results. One common error is misformulating null and alternative hypotheses, which can lead to testing the wrong assumptions.
Confusing qualitative and quantitative methods can produce irrelevant or misleading outcomes. Without clear objectives, you might test variables that aren’t meaningful, wasting time and resources. Incorporating appropriate research design can help prevent this issue.
Incorrectly identifying independent and dependent variables can also skew results. Ignoring the importance of effect size leads to overemphasizing statistical significance without considering practical relevance.
Additionally, misinterpreting p-values, calculating critical values incorrectly, or making arithmetic mistakes can compromise your analysis. Failing to contemplate sample size, confounding variables, or proper correction methods further increases errors.
Avoid these pitfalls by planning carefully, understanding your data, and applying appropriate statistical principles. Incorporating sustainable practices in your research approach can enhance the reliability and impact of your findings.
Real-World Examples of Hypothesis Testing
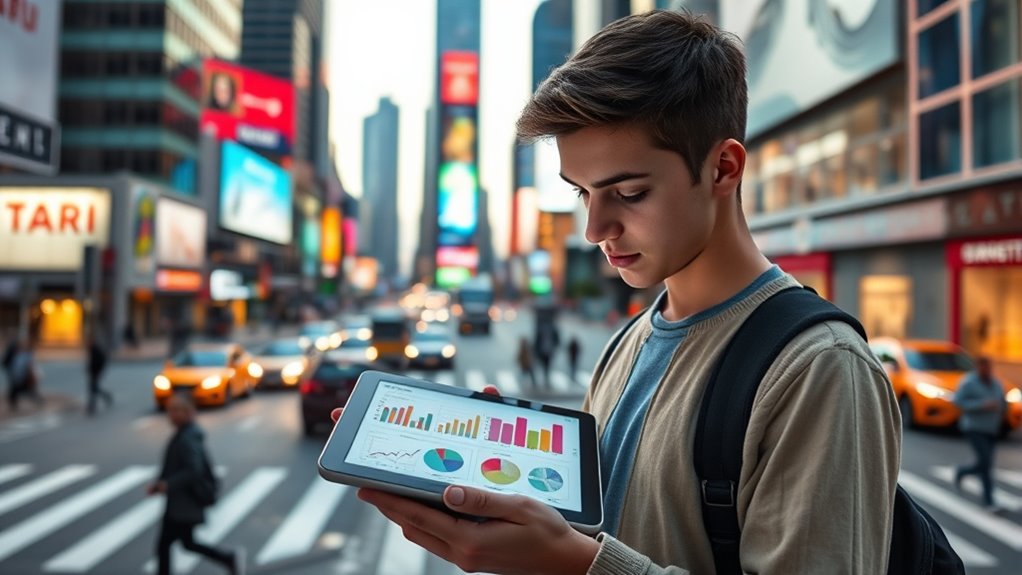
Hypothesis testing plays an essential role in real-world decision-making across various fields.
In health and medicine, it’s used in clinical trials to evaluate new drugs, such as blood pressure or cholesterol-lowering medications, to confirm if they meet efficacy standards. Vaccines are tested for effectiveness, and disease incidence studies help identify risk factors.
In environmental science, it assesses pollution’s impact on ecosystems, like fish populations, and evaluates the effects of chemical spills. Climate change and air quality studies rely on hypothesis tests to analyze trends and standards.
In industry, it guarantees manufacturing quality, verifies product improvements, and predicts maintenance needs.
These examples highlight how hypothesis testing guides crucial decisions, ensuring reliability and effectiveness across diverse sectors. Additionally, the rigorous methodology behind hypothesis testing helps validate results and reduce errors, making it an indispensable tool in scientific research and practical applications.
Frequently Asked Questions
How Does Sample Size Affect Hypothesis Test Accuracy?
You should know that larger sample sizes improve the accuracy of hypothesis tests. They help you get closer to the true population parameters and reduce variability in your estimates.
With bigger samples, your confidence intervals narrow, making your results more reliable.
Smaller samples, on the other hand, can lead to misleading conclusions because of higher variability.
What Are Common Signs of Misinterpreting P-Values?
Imagine you’re steering a river, mistaking ripples for a storm. When you misinterpret p-values, you might think they’ve shown you a real effect, but they’re just ripples, not storms.
Signs include believing a low p-value proves a hypothesis is true, or that statistical significance equals real-world importance. You might also ignore effect size, thinking p-values tell a whole story, leading to overconfidence and flawed conclusions.
When Should Nonparametric Tests Be Preferred Over Parametric Ones?
You should prefer nonparametric tests when your data aren’t normally distributed, or when you’re working with ordinal, nominal, or small sample sizes.
They’re ideal if your data contain outliers or don’t meet the assumptions required for parametric tests. These tests are more flexible, robust, and don’t rely on specific distribution assumptions, making them a reliable choice in diverse situations where parametric methods might give misleading results.
How Do I Handle Multiple Hypothesis Testing Issues?
Imagine you’re steering a busy marketplace with many stalls, each promising a special deal. Handling multiple hypothesis tests is like managing those stalls—you risk falling for false bargains.
To avoid false alarms, you use tools like the Bonferroni correction or FDR control, which act like a trusted guide, helping you focus on genuine deals while minimizing false positives. This way, you make smarter, more reliable decisions.
What Ethical Considerations Are Involved in Hypothesis Testing?
When considering ethical aspects in hypothesis testing, you should prioritize informed consent, ensuring participants understand the study.
Maintain data privacy and avoid coercion or manipulation.
Transparently report all results, including negative or insignificant findings, and clearly explain your methodology.
Be cautious about overstating conclusions, distinguish correlation from causation, and prevent misinterpretation.
Finally, adhere to professional guidelines, handle data responsibly, and seek institutional review to uphold ethical standards throughout your research.
Conclusion
Remember, hypothesis testing is your compass in data exploration—guiding you through uncertainty like a lighthouse in fog. Every step you take, from setting hypotheses to interpreting results, shapes your understanding. Think of your data as a seed; with careful testing, it blooms into insights. Trust the process, avoid common pitfalls, and let your curiosity be the wind that drives your journey toward clarity and discovery.