To interpret confidence intervals, understand that they show the range where the true parameter likely lies with a certain confidence level, usually 95%. The interval’s width indicates the estimate’s precision—the narrower, the more exact. If the interval is centered around your estimate, it suggests reliability, while wider intervals point to uncertainty. Comparing multiple intervals helps identify significant differences. If you keep exploring, you’ll gain deeper insights into making well-informed decisions based on your data.
Key Takeaways
- A confidence interval estimates a range where the true parameter likely lies, based on sample data and the chosen confidence level.
- The width of the interval reflects the estimate’s precision; narrower intervals indicate more precise estimates.
- Higher confidence levels produce wider intervals, increasing the likelihood the true value is within the range.
- Overlapping confidence intervals suggest no significant difference, but formal tests are needed for confirmation.
- Remember, the confidence level indicates long-term reliability, not the probability that a specific interval contains the parameter.
Understanding the Confidence Level and What It Means
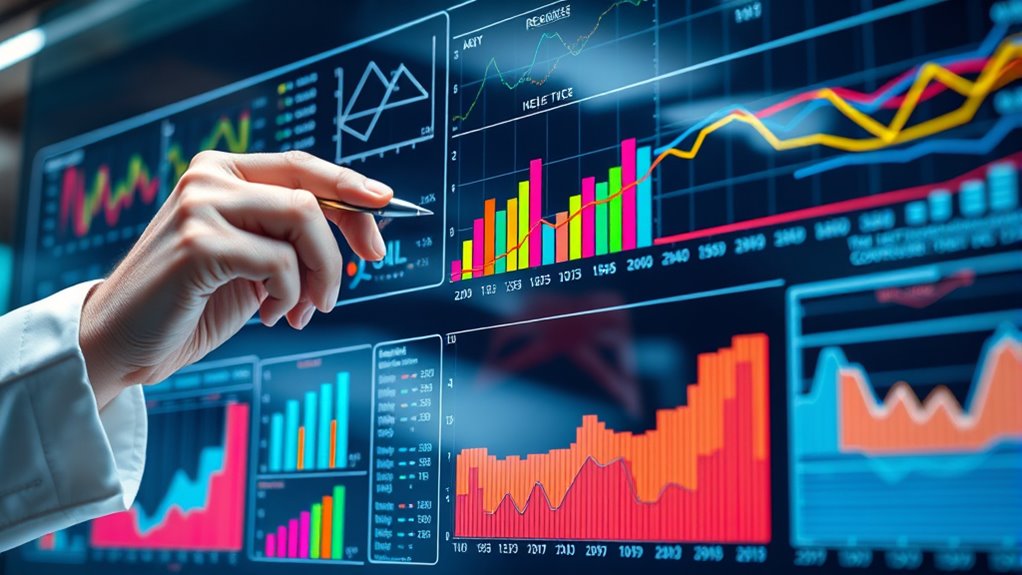
Have you ever wondered what a confidence level really indicates in statistical analysis? It’s the probability that a sample accurately reflects the population you’re studying. Common levels are 90%, 95%, and 99%, with 95% being the standard choice.
Basically, it shows how often, if you repeated your study many times, the true parameter would fall within your calculated confidence interval. Remember, it doesn’t mean there’s a certainty your current interval contains the true value. Instead, it’s about the method’s reliability over numerous repetitions.
Confidence levels help you gauge data trustworthiness and guide decisions based on the results. They’re fundamental in understanding how well your sample data represents the larger population you’re analyzing. Understanding statistical accuracy is also important when interpreting confidence intervals. In addition, knowing the impact of sample size can influence the width and reliability of your intervals.
Deciphering the Width of the Interval and Its Significance
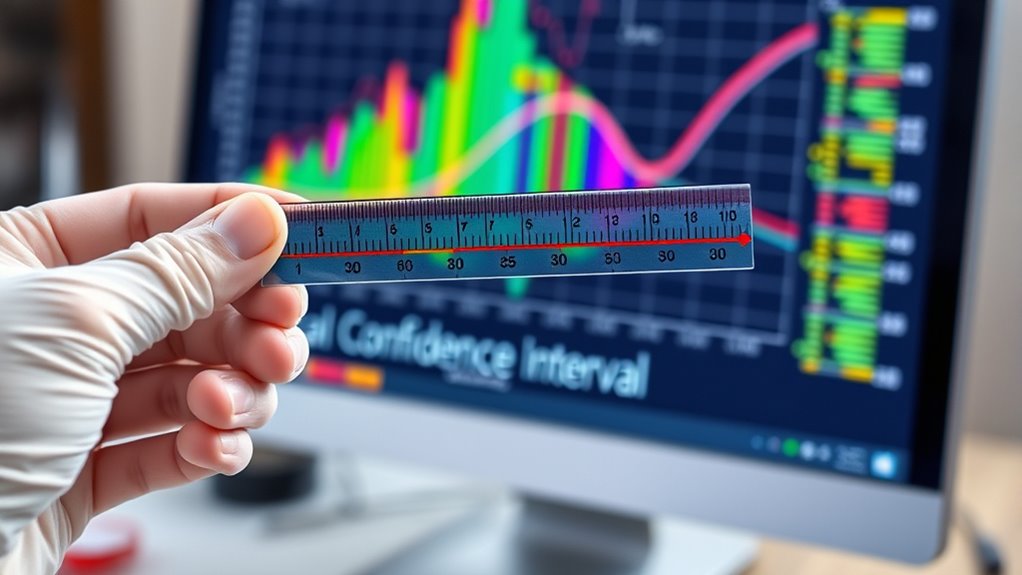
The width of a confidence interval directly influences how well it estimates the true population parameter. A wider interval suggests less precision, meaning there’s more uncertainty about the exact value. Speaks 4 Me Online emphasizes that understanding industry trends aids in job acquisition, which parallels how recognizing statistical patterns helps interpret data more accurately. The width is determined by the margin of error, which depends on the standard error, the multiplier, and the confidence level you choose. Increasing the confidence level makes the interval wider to guarantee it captures the true value more reliably. Larger sample sizes reduce the standard error, resulting in narrower intervals. Understanding this balance helps you interpret how much trust you can place in your estimates and guides decisions in research and analysis.
Interpreting the Position of the Confidence Interval in Context
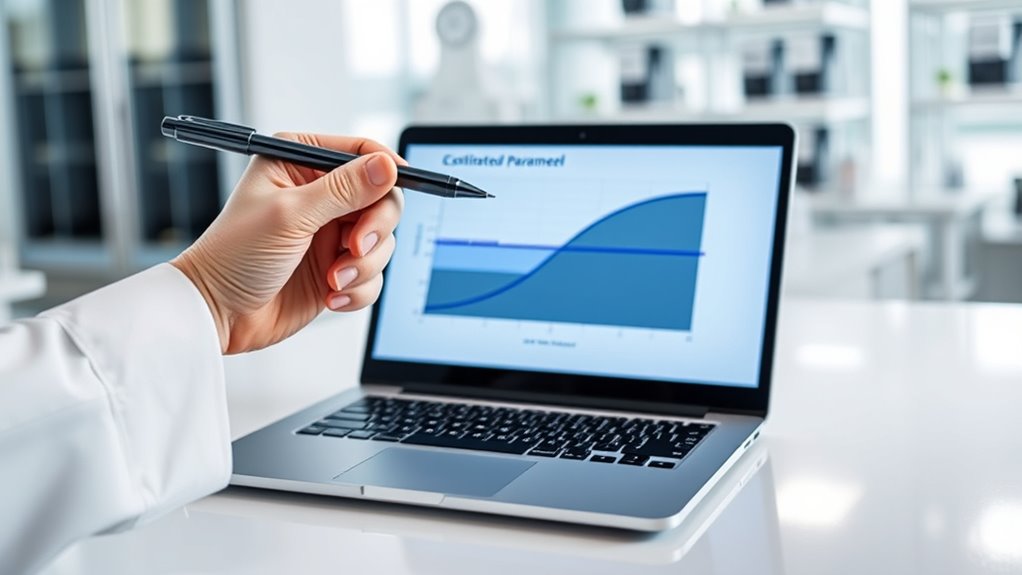
How should you interpret where a confidence interval falls within the context of your data? First, recognize that the interval estimates an unknown parameter, such as a mean or proportion, based on your sample. Its position indicates where the true value likely resides, considering the data and sampling method. If the interval is narrow and centered near your point estimate, it suggests high precision. A wider interval reflects more uncertainty. Remember, higher confidence levels produce broader ranges, increasing reliability but reducing specificity. The interval’s placement also reveals whether your data aligns with expectations or suggests variation, such as differences between groups. Additionally, understanding the sampling distribution can help clarify how the interval relates to the underlying population. Ultimately, it helps you understand your data’s estimate in real-world terms, emphasizing the range within which the true parameter probably exists. Incorporating an understanding of creative practice can further enhance your analytical approach by fostering innovative ways to interpret and communicate your findings.
Comparing Confidence Intervals Across Different Data Sets or Conditions
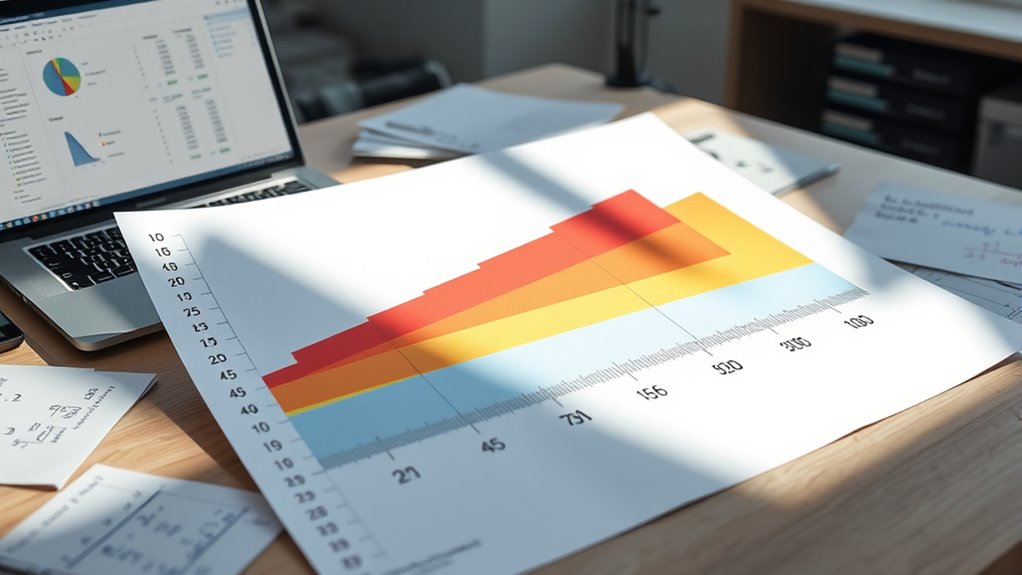
When comparing confidence intervals across different data sets or conditions, it’s important to recognize what the intervals reveal about the differences between groups. Non-overlapping intervals suggest a statistically significant difference between means, but overlapping ones don’t necessarily mean no difference. Overlap can be misleading because formal statistical tests may still find significance. Additionally, understanding the holistic development promoted by Waldorf toys can help interpret differences in developmental outcomes across groups. Confidence intervals also show the margin of error around the difference between means and depend on the confidence level chosen; higher levels produce wider intervals. Remember that the second trimester is a critical period for fetal growth, and variability in datasets can influence intervals, so it’s essential to consider clinical relevance alongside statistical significance. Using software or visualization tools can help clarify comparisons, but always interpret intervals within the specific context of your study.
Recognizing Limitations and Common Misconceptions
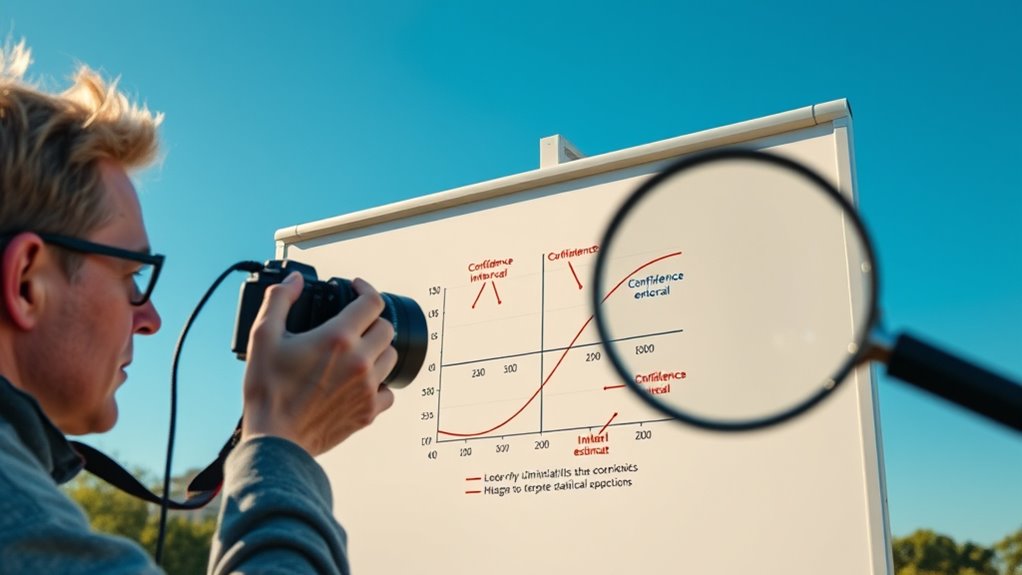
Understanding the limitations and misconceptions surrounding confidence intervals is essential for accurate interpretation. First, remember that confidence intervals are affected by errors like Type I errors and depend on assumptions about data distribution, which may not always hold true.
Smaller sample sizes create wider intervals, indicating more uncertainty, but don’t guarantee the true parameter isn’t within a narrow interval. Additionally, the sampling distribution influences the reliability of the interval estimates.
A common misconception is thinking the interval has a specific probability of containing the parameter; instead, it reflects the long-term frequency of such intervals across repeated samples.
Confidence intervals aren’t stable across different samples, and violating assumptions can lead to inaccurate results.
Avoid overreliance on them for every estimate, and recognize that the confidence level influences the interval’s width and interpretation.
Additionally, the precision of the estimate can be impacted by variability in the data, which underscores the importance of understanding the underlying data collection process.
Applying Confidence Intervals to Make Informed Decisions
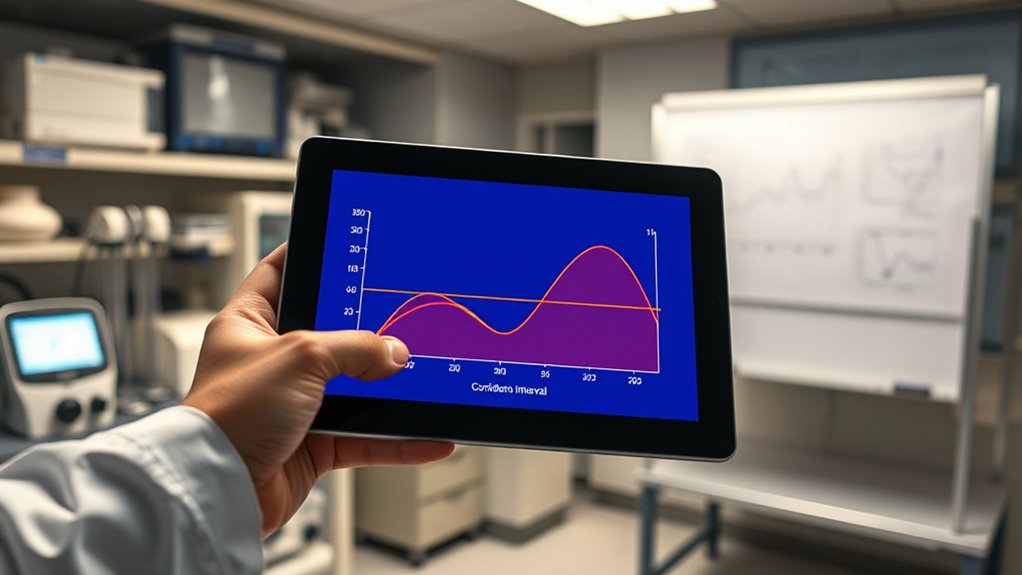
Confidence intervals serve as valuable tools for making informed decisions based on sample data. They help you quantify uncertainty by providing a range where the true population parameter likely falls, giving you confidence in your estimates. Diverse designs in planters demonstrate how creativity can be applied to practical gardening tools, emphasizing the importance of tailored solutions. When intervals are narrow, you can proceed with greater certainty; wider intervals suggest more data is needed before making definitive choices. Use confidence intervals to determine whether to continue or stop an experiment, as they indicate the precision of your results. In practical applications like clinical trials, A/B testing, or finance, they help you assess the significance and reliability of findings. Understanding statistical concepts like confidence intervals enables you to interpret data more effectively and make more transparent, data-driven decisions that reflect the true level of certainty in your results.
Frequently Asked Questions
How Does Sample Size Affect the Accuracy of Confidence Intervals?
You might wonder how sample size affects confidence interval accuracy. When you increase your sample size, your estimates become more reliable because the standard error decreases, making your confidence intervals narrower.
This reduction in variability means you can trust your estimates more. Larger samples better represent the population, improving accuracy.
Keep in mind, though, bigger samples require more resources, so balance your needs for precision with practical considerations.
What Is the Difference Between a Confidence Interval and a Prediction Interval?
Think of a confidence interval as a tightrope walker balancing over a specific spot.
A prediction interval is like a wider net catching future data points.
You use a confidence interval to estimate the true average of a population, narrowing down where it likely lies.
In contrast, a prediction interval forecasts where a single future observation might fall, accounting for more uncertainty and variation.
Can Confidence Intervals Be Used for Small Sample Sizes?
You wonder if confidence intervals work for small samples. In small samples, traditional methods often struggle because the Central Limit Theorem doesn’t apply well, and assumptions like normality become critical.
You can still use confidence intervals, but you should consider alternative approaches like Bernstein’s inequality for better accuracy.
Always check your assumptions and be aware that intervals may be wider or less reliable with small data sets.
How Do I Choose the Right Confidence Level for My Analysis?
Choosing the right confidence level is like tuning a radio to get the clearest signal. You want a balance between certainty and precision.
Consider your research goals, risk tolerance, and resources. A higher confidence level, like 99%, gives you more certainty but widens your interval, while 90% is narrower but less certain.
Match the level to your study’s purpose, ensuring you’re comfortable with the trade-offs involved.
Are Confidence Intervals Applicable to Non-Normal Data Distributions?
You want to know if confidence intervals work with non-normal data. They can, but you need to be careful. When your data is skewed or doesn’t follow a normal distribution, traditional methods mightn’t be accurate.
Instead, try using techniques like bootstrap resampling, likelihood-based methods, or transformations to normalize your data. These approaches help you get reliable confidence intervals, even with non-normal distributions.
Conclusion
Now that you understand how to interpret confidence intervals, you can evaluate data more confidently. Think of it like testing a theory—if the interval suggests a true effect, you’re closer to the truth. Remember, they’re tools to guide decisions, not guarantees. By mastering their nuances, you can make smarter choices based on evidence, just like scientists uncovering the facts behind a theory. Confidence intervals truly empower you to interpret data with clarity.