Correlation means two variables change together, but it doesn’t prove one causes the other—there could be other factors involved. Causation, on the other hand, shows a direct cause-and-effect relationship where one variable influences the other. It’s easy to mistake correlation for causation, but understanding the difference is key for interpreting data accurately. If you want to learn how to recognize these distinctions and avoid common mistakes, keep exploring further.
Key Takeaways
- Correlation indicates a statistical relationship where two variables move together but do not imply one causes the other.
- Causation means one variable directly influences or produces a change in another variable.
- Two variables can be correlated due to coincidence, confounding factors, or underlying causes, not necessarily causality.
- Establishing causation requires controlled experiments and evidence that changes in one variable lead to changes in the other.
- Mistaking correlation for causation can lead to false conclusions and misguided decisions.
Understanding the Basic Concepts of Correlation and Causation
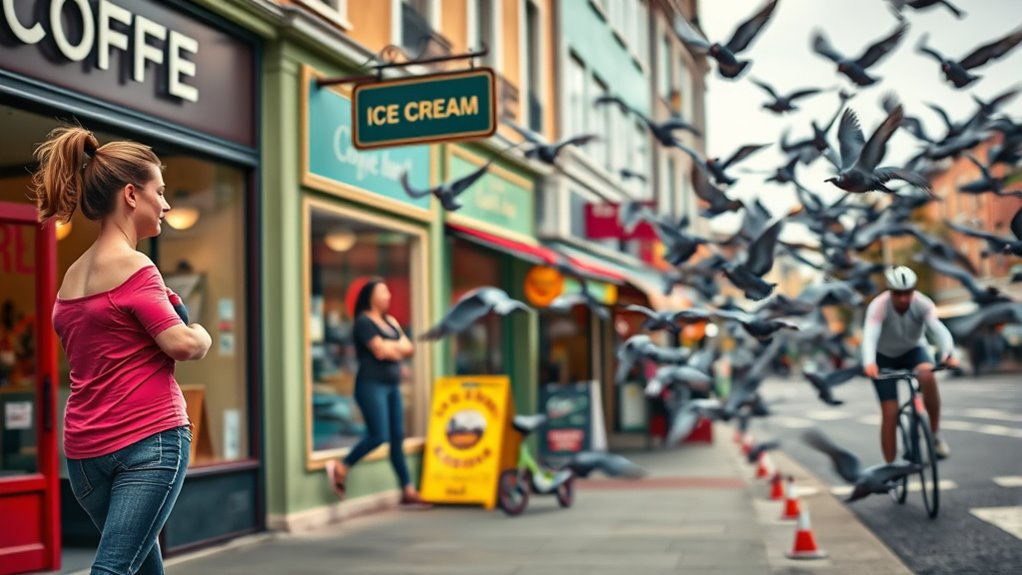
To understand the difference between correlation and causation, it’s important to recognize that correlation describes a statistical relationship where two variables tend to change together, either increasing or decreasing simultaneously, without implying that one causes the other.
When two variables are correlated, they may move in the same or opposite directions, but this doesn’t mean one influences the other directly. Correlation is measured by a number that shows the strength and direction of the relationship.
In contrast, causation means one variable directly affects another, creating a cause-and-effect link. Just because two things happen together doesn’t mean one causes the other.
Recognizing this distinction helps you avoid mistaken assumptions and interpret data more accurately. Understanding the role of content quality and authority in data interpretation can further clarify why some correlations may be misleading or coincidental. Additionally, it is essential to consider other factors like confounding variables, which can influence both correlated variables without a direct causal relationship.
How to Identify Correlation in Data
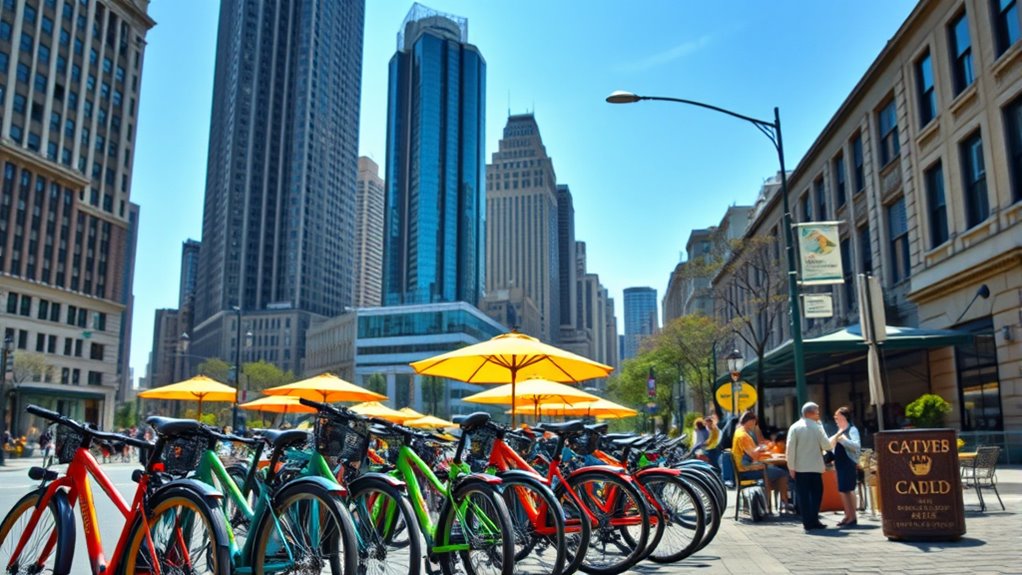
Want to determine if two variables are related? Start by examining scatter plots.
Look for patterns: a clear upward or downward trend suggests positive or negative correlation. If points form a straight line, it indicates a linear relationship, often linked to Pearson’s correlation.
Identify clear upward or downward trends to spot positive or negative correlations effectively.
If the pattern is monotonic but curved, Spearman’s rank correlation may be appropriate.
For categorical data, use heatmaps of correlation matrices or Cramer’s V to identify associations.
Numerical values of correlation coefficients help interpret strength: near ±1 means a strong relationship, while close to 0 indicates little to no correlation.
Keep in mind data should be paired, free of extreme outliers, and ideally normally distributed for accurate analysis.
Larger sample sizes and visual tools make it easier to spot meaningful correlations.
Additionally, understanding the Bedroom environment can influence the quality of data collection in observational studies related to human behavior. Recognizing how contextual factors impact data collection can improve the accuracy of correlation analysis.
The Challenges in Establishing Causation
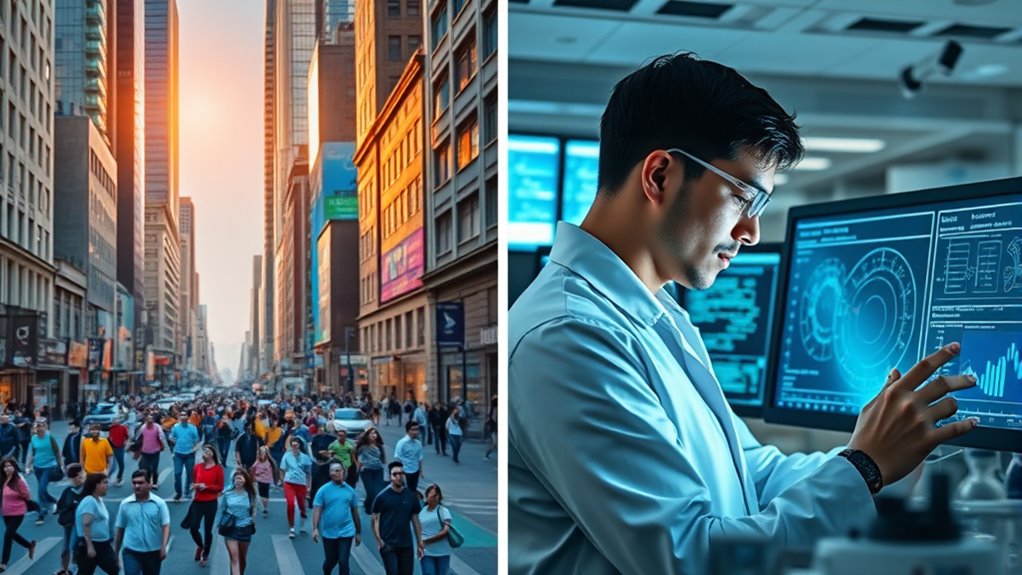
Establishing causation in research is inherently challenging because numerous factors can interfere with clear conclusions. Study design limitations, like lack of realism and ethical constraints, restrict manipulating variables. Small sample sizes increase costs and reduce reliability, while complex systems with many interacting factors make isolating causes difficult. Additionally, the presence of recurring patterns or behaviors, such as relationship cycles, can complicate interpretations of cause and effect. Confounding variables, often unmeasured or unknown, can distort relationships, leading you astray if not properly adjusted for. Biases from data collection—selection, information, or attrition—further muddy the waters. Moreover, understanding mechanisms involves steering through complex causal chains and environmental influences, which are costly and resource-intensive. Research design plays a crucial role in addressing these challenges by helping to control variables and establish clearer causal links. Timing issues, such as establishing that the cause precedes the effect, add another layer of difficulty.
All these factors combine, making it hard to confidently prove causation rather than mere correlation.
Real-World Examples Demonstrating the Difference
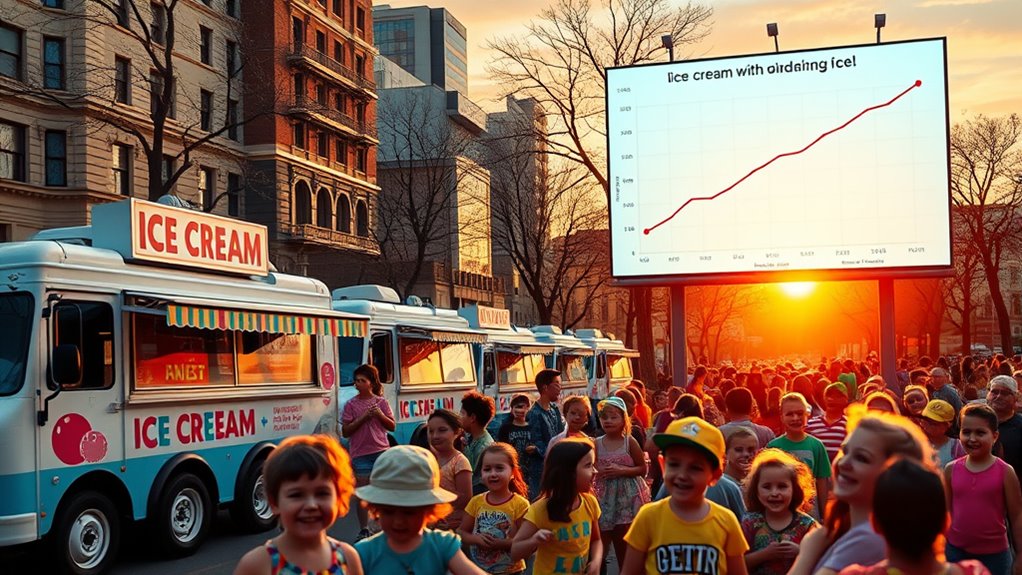
Understanding the difference between correlation and causation becomes clearer when you look at real-world examples. For instance, ice cream sales and shark attacks are correlated, but weather explains both—hot days increase ice cream purchases and swimming, which raises shark attack risks.
Similarly, high temperatures lead to more ice cream consumption and sunburns, but temperature itself isn’t the direct cause of either. Recognizing confounding variables helps clarify these relationships.
Economic data shows that the number of high school graduates and pizza sales rise together because of population growth, not because graduates cause pizza sales.
These examples highlight how external factors, like weather or demographics, influence seemingly related variables without one directly causing the other. Recognizing these patterns helps you avoid false assumptions about causation based solely on correlation.
Understanding the concepts of correlation and causation is essential for accurate data interpretation and making informed decisions.
Common Mistakes When Interpreting Relationships Between Variables
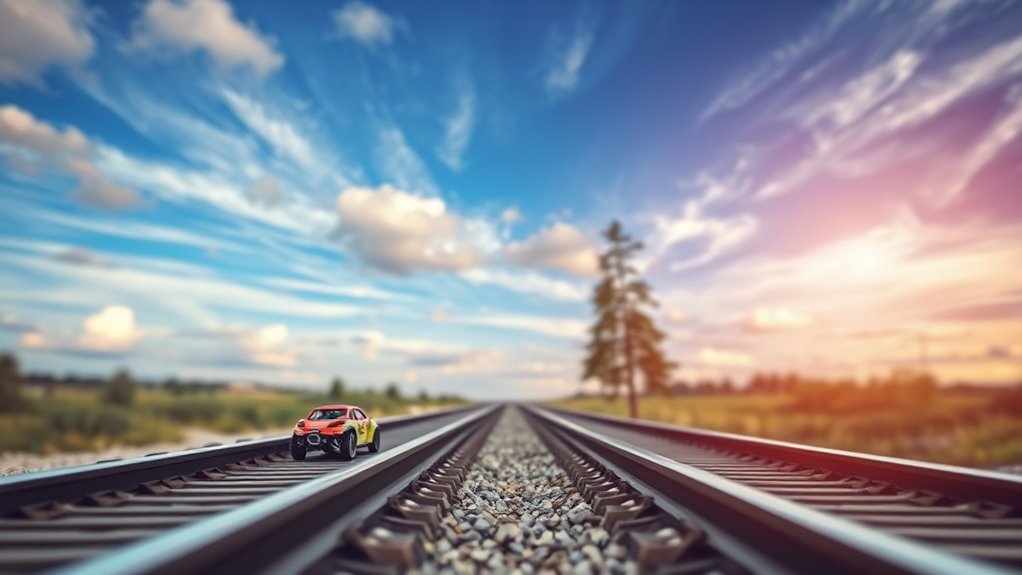
Interpreting the relationships between variables often leads to mistakes if you’re not careful about the details. One common error is ignoring data types; treating categorical data as numerical can lead to invalid analyses.
Ignoring variable data types can lead to invalid analysis and misinterpretation.
You also might assume relationships are linear without checking for non-linear patterns, which can mislead you. Outliers can heavily skew correlation coefficients, making relationships seem stronger or weaker than they truly are. Being aware of cookie categories helps understand how different types of data influence analysis and interpretation.
Failing to identify subgroups within your data can produce false overall correlations. Remember, correlation doesn’t imply causation—just because two variables move together doesn’t mean one causes the other.
Additionally, overlooking the influence of confounding variables can result in incorrect conclusions about the nature of the relationship between variables.
Misinterpreting the strength of correlation coefficients or overrelying on statistical significance without context can lead you astray. Always consider the broader picture and potential hidden factors.
Frequently Asked Questions
Can Two Variables Be Causally Related Without Showing Correlation?
Yes, you can have a causal relationship without showing a clear correlation. This happens when the relationship is complex, non-linear, or masked by other factors.
You might also miss the correlation because of small or biased samples or limitations in statistical methods.
In such cases, causation exists, but it’s not easily detectable through simple correlation analysis, requiring more advanced or controlled experiments to reveal the true relationship.
How Can Confounding Variables Affect Correlation and Causation?
Confounding variables can distort your understanding of relationships by creating false correlations, hiding real ones, and misleading causal claims.
They influence both variables, making unrelated factors seem linked or genuine effects appear absent.
You might overestimate or underestimate effects, attributing causes where none exist.
To avoid this, you need to identify, measure, and control for confounders through thoughtful research design, statistical adjustments, and careful data collection.
What Statistical Methods Are Best for Establishing Causation?
You want to know which statistical methods best establish causation. You should focus on techniques like randomized controlled trials, which directly manipulate variables and control confounders.
Propensity score matching and instrumental variable analysis help in observational studies by balancing covariates and addressing confounding.
Difference-in-differences compare groups over time, while structural equation modeling explores complex causal pathways.
These methods strengthen your ability to infer causality beyond mere associations.
Is It Possible for a Strong Correlation to Be Purely Coincidental?
Is a strong correlation just a coincidence waiting to be uncovered? Yes, it’s possible. Sometimes, random chance or lurking variables craft seemingly meaningful links, like ice cream sales and drowning incidents, driven by summer heat.
You must be cautious, as these correlations often mask confounding factors. Without thorough analysis, you risk mistaking coincidence for causation, falling into the trap of false connections that look convincing but lack real substance.
How Do Temporal Sequences Help Differentiate Causation From Correlation?
You want to know how temporal sequences help distinguish causation from correlation. When you establish that the cause happens before the effect, you strengthen the case for causation.
Temporal sequences guarantee the chronological order is correct, preventing reverse causation. By tracking events over time, you can see which came first, making it clearer whether a true causal link exists or if it’s just a coincidence.
Conclusion
Now that you understand the difference between correlation and causation, you realize how easy it is to jump to conclusions. But what if there’s more beneath the surface? The true challenge lies in uncovering the hidden links and avoiding common pitfalls. Will you recognize the clues that reveal causation or be misled by mere correlations? Stay curious—next, you’ll discover how to dig deeper and uncover the real stories behind the data.