To calculate the mean in statistics, gather your data from reliable sources and verify it’s clean and organized. Sum all the data points, then count how many observations there are. Divide the total sum by this number to find the average. Be aware of outliers or skewed data, as they can affect the mean’s accuracy. If you want to learn more about interpreting and visualizing the mean, keep exploring further.
Key Takeaways
- Sum all data points using a calculator, software, or manually to find the total sum (Σxᵢ).
- Count the number of data points (n) in your dataset accurately.
- Divide the total sum by the number of data points (n) to compute the mean.
- Organize data clearly before calculation to minimize errors.
- Use visual tools like histograms or box plots to interpret how the mean represents the data’s center.
Understanding the Concept of Mean
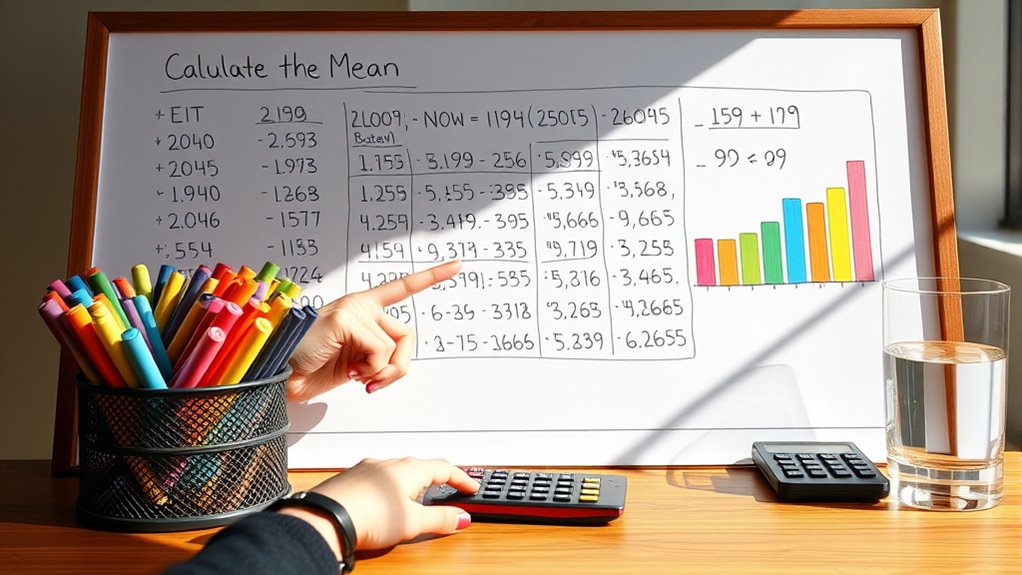
Have you ever wondered what the average of a set of numbers really tells you? The mean is a key measure of central tendency, giving you a single value that represents the middle point of your data. It’s calculated by adding all the data points together and dividing by how many there are. Think of it as the balance point of your dataset.
The mean helps summarize large amounts of information quickly and clearly. It’s especially useful because it’s simple to compute and provides a concise overview. However, be aware that outliers—extreme values—can skew the mean, making it less representative of the overall data.
Understanding the mean helps you interpret data more effectively, whether in everyday decisions or complex statistical analysis.
Gathering Data for Calculation
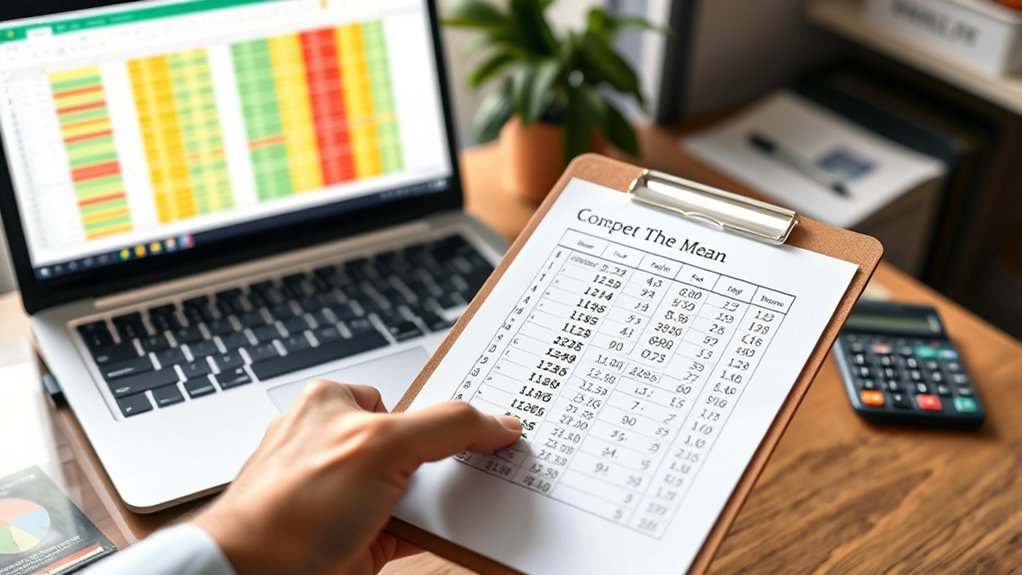
Gathering accurate data is essential for calculating the mean effectively. To do this, you need reliable sources like surveys, which gather quantitative data on consumer preferences, or interviews for detailed insights. Focus groups can reveal opinions from multiple participants, while transactional tracking helps identify patterns from real-world transactions. Additionally, understanding the effectiveness of eye patches can help interpret the significance of your data findings. Employing behavioral observations allows you to record behaviors without interference. Use data collection tools such as questionnaires and online forms for structured data, or web scraping tools and social media monitoring to gather online insights. Employing professional cleaning services can ensure data accuracy when collecting from physical environments, especially in settings like mechanic shops or cleaning services. Recognizing the importance of diversification in your data sources can help improve the reliability of your analysis. Ensuring the data collection process adheres to privacy policies helps maintain ethical standards and builds trust with participants. Depending on your goal, you might collect primary data directly or utilize secondary data from existing sources. Ensure your data is relevant, consistent, and exhaustive to enable accurate calculation of the mean later on. Proper data gathering forms the foundation for meaningful statistical analysis.
Summing the Data Values
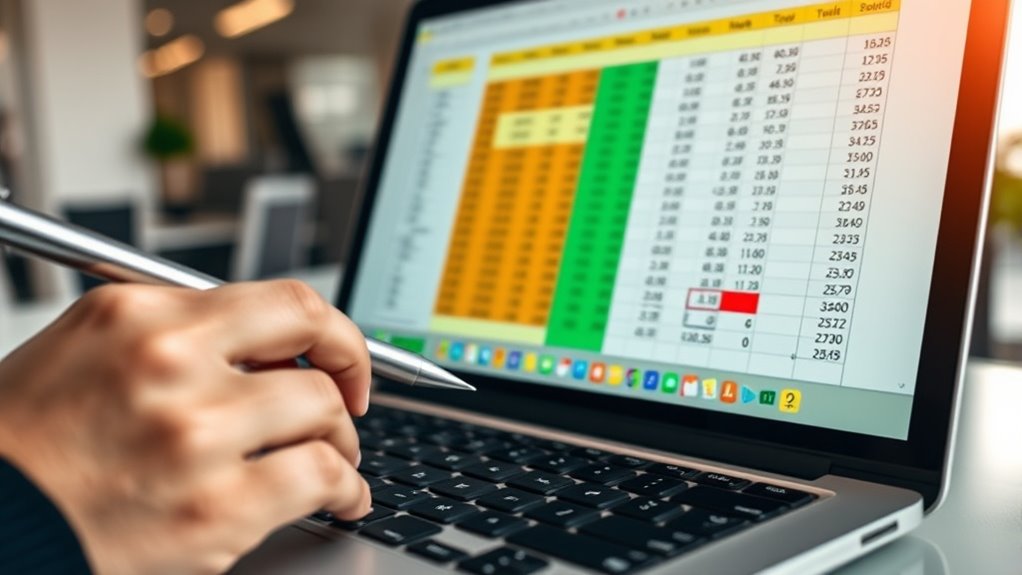
To accurately calculate the mean, you first need to find the sum of all data values in your dataset. This involves adding each number together, whether manually or using tools like calculators or software. Forsale 100 is an example of a value that might be included in your dataset, emphasizing the importance of accurate summing for meaningful analysis. The total sum, often represented by the notation ( sum_{i=1}^{n} x_i ), is essential for the calculation. Using a calculator or spreadsheet can save time and reduce errors, especially with larger datasets. Summing data is also foundational for other analyses, such as calculating variance or standard deviation. When you sum your data, you’re consolidating all values into a single number that reflects the total of your dataset. This step is crucial for ensuring data accuracy and consistency throughout your statistical analysis. Additionally, understanding how to properly calculate the sum helps in maintaining data integrity throughout your statistical analysis. Proper summation ensures that subsequent calculations like the mean are based on reliable data.
Counting the Number of Data Points
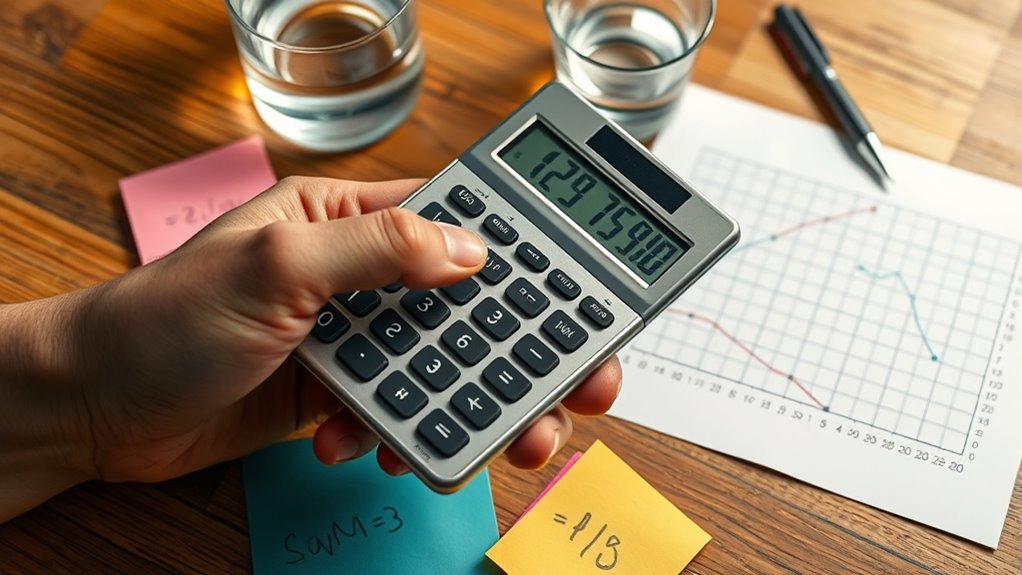
Counting the number of data points is a key step in calculating the mean because it determines how many values you’ll be averaging. You need to know this count to divide the total sum accurately. Additionally, understanding the bedroom environment can influence how you interpret data related to sleep patterns or habits. For count data, which includes whole numbers like the number of species or sunny days, counting can be done manually or with software. Larger datasets are typically counted using statistical tools, ensuring efficiency and accuracy. Remember, the size of your dataset impacts the reliability of your mean; bigger samples generally provide more precise estimates due to the law of large numbers. Ensuring your data is clean and error-free before counting helps maintain data integrity and accuracy. Properly data collection methods are essential to obtain reliable results, especially in large datasets. Using tools such as headphones can help improve focus during data analysis, reducing errors. Precise counting is essential because any mistake can skew your results and lead to incorrect conclusions.
Applying the Basic Mean Formula
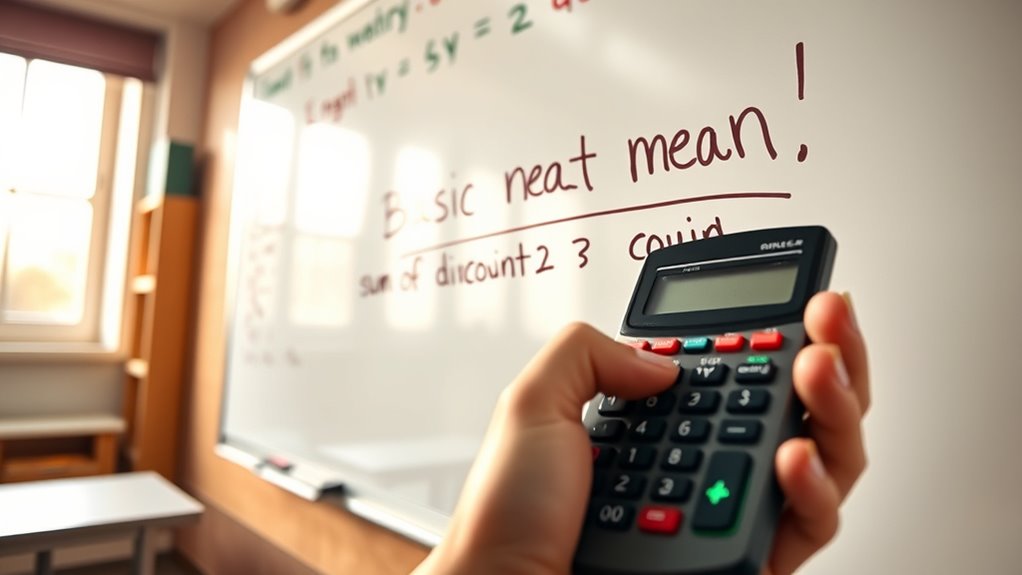
Applying the basic mean formula involves straightforwardly calculating the average by dividing the total sum of data values by the number of observations. You first add up all the data points to find the total sum, represented as ( Sigma x_i ). Then, you divide this sum by the total number of data points, ( n ). This process gives you the arithmetic mean, which is the most common measure of central tendency. Understanding how to accurately perform this calculation is essential for analyzing data effectively, especially when working with statistical measures. The formula remains the same whether you’re working with raw data or grouped data, although different methods may be used for grouped data. Additionally, ensuring that data is properly collected and organized helps improve the accuracy of the mean calculation. Proper data management techniques, such as cleaning and validating datasets, further contribute to precise results. Being aware of potential outliers in the data can also help prevent skewed averages and improve the reliability of your analysis. Recognizing data variability is important for interpreting the mean accurately.
Calculating Mean From Raw Data
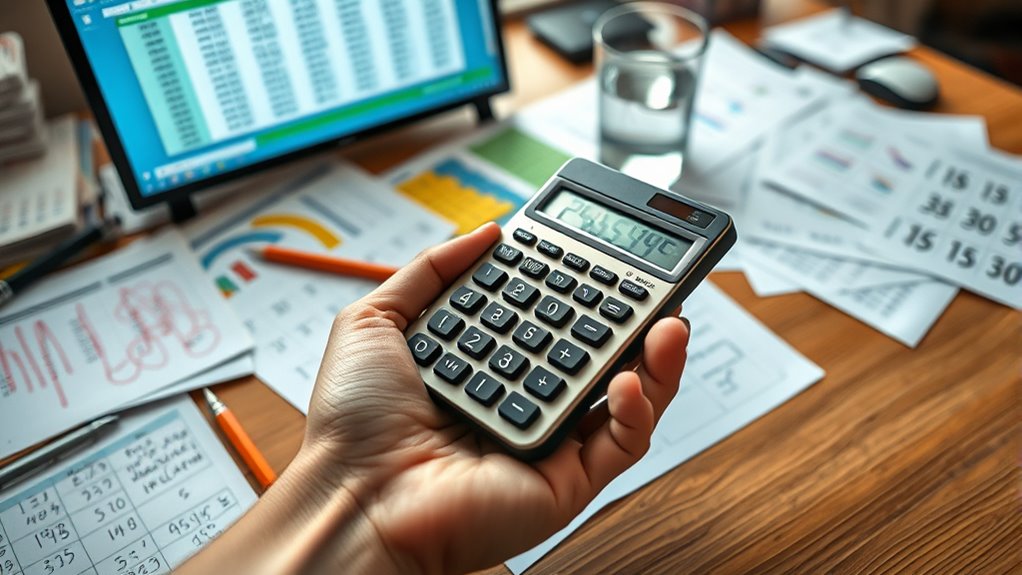
Calculating the mean from raw data involves straightforward steps that start with organizing your data points. First, list all your data values clearly, either vertically or horizontally, whichever is more convenient. Next, use a calculator to sum all the data points accurately, ensuring no values are missed or duplicated. Count the total number of data points to determine your dataset’s size. Then, divide the total sum by this number to find the mean. Remember to verify your calculations to avoid errors. If needed, round the result for simplicity, but keep in mind that precise values often provide better insights. Additionally, understanding the role of the mean in summarizing data helps in interpreting the overall trend effectively. Recognizing how performance tuning can optimize system efficiencies enhances your analysis of data outcomes. Being aware of common family photoshoot fails can also help in preparing for unexpected issues during data collection or presentation. Finally, interpret what the mean represents in your context, such as average spending or hours worked, to derive meaningful conclusions from your raw data. Understanding the cultural significance of data interpretation can deepen your insights and improve decision-making processes. Moreover, considering the comprehensive approach used in holistic methods can help in gaining a more complete understanding of your dataset.
Using Grouped Data for Mean Calculation
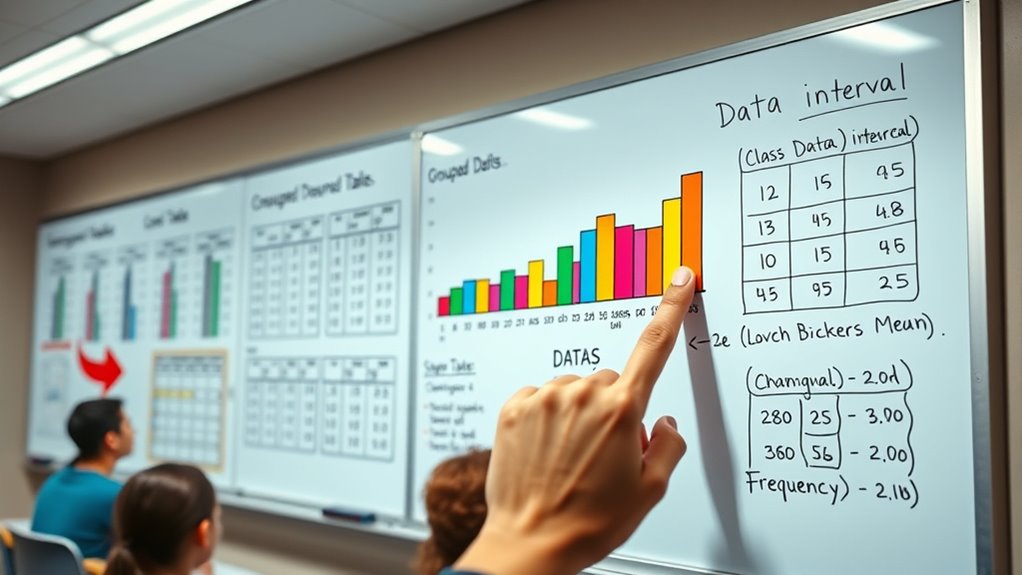
When working with grouped data, estimating the mean involves using the midpoints of each class interval to represent all observations within that group. You first find the midpoint by averaging the lower and upper limits of each interval. A new sentence can be added here: This approach simplifies complex datasets and provides a practical way to analyze data distribution in large datasets. Next, identify the frequency of observations in each group. Then, multiply each midpoint by its corresponding frequency to get the total for that interval. Sum all these products and divide by the total number of observations to estimate the mean. Keep in mind, this method assumes all data points within a group are at its midpoint, so the result is an estimate. Using grouped data simplifies complex datasets and helps reveal general trends, but it sacrifices some detail and precision for easier analysis. For example, TikTok trends can be analyzed using grouped data to identify overall patterns despite individual variability. Additionally, understanding the law of attraction can enhance your mindset when interpreting data trends and making positive changes based on insights.
Handling Outliers and Skewed Data
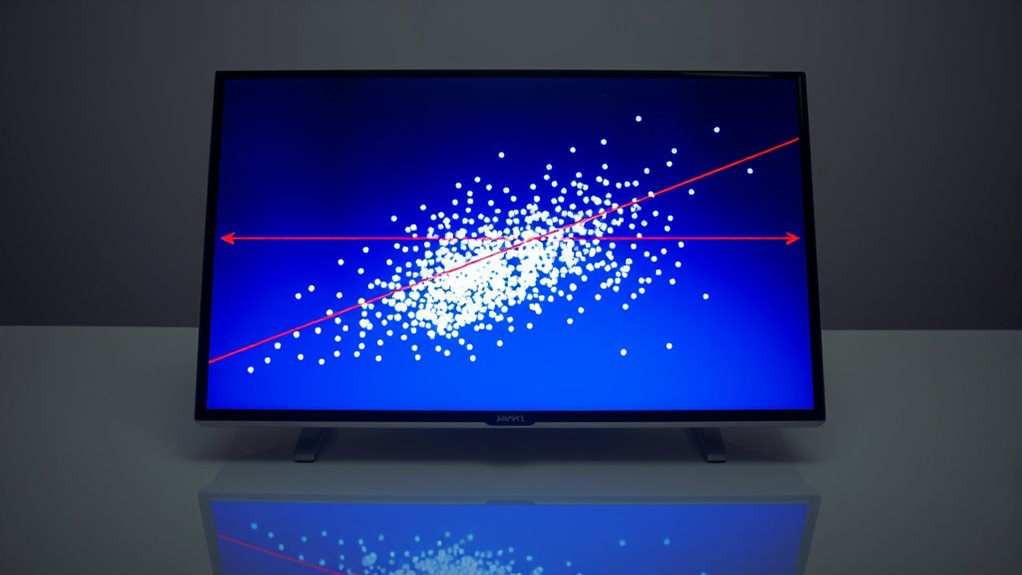
Outliers and skewed data can substantially affect the accuracy of your statistical analysis, especially when calculating the mean. Outliers are data points that are far from other observations and can distort the average.
Outliers and skewed data can distort the mean, impacting your analysis accuracy.
To identify them, you can use methods like the z-score, where values with scores above 3 or below -3 are outliers, or the IQR method, which flags points more than 1.5*IQR from quartiles. Visual tools like box plots help you spot outliers quickly.
To handle these, you might remove errors, transform data, or use robust statistics less sensitive to outliers. In skewed distributions, transformations like the log can make the data more normal.
These strategies help guarantee your mean remains a reliable measure of central tendency.
Interpreting the Mean in Context
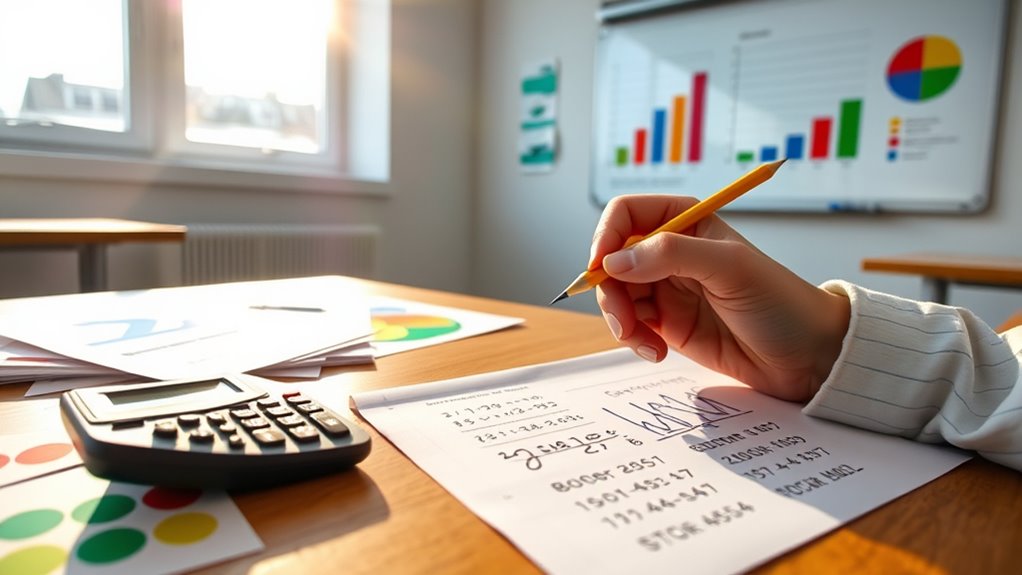
Understanding the mean’s value isn’t enough; you also need to interpret what it tells you within the context of your data. The mean reveals the average but can be misleading if you ignore factors like distribution shape or outliers.
For example, in income data, a high mean may be skewed by a few wealthy individuals, making it unrepresentative of most people. To interpret meaningfully, consider how the mean relates to other measures like the median or standard deviation.
Recognize its limitations in skewed or outlier-rich datasets. Context helps you decide whether the mean accurately reflects your data’s typical values or if alternative measures provide better insights.
Ultimately, correct interpretation guides better decisions and prevents misjudgments based solely on numerical averages.
Visualizing Data Distributions With Mean
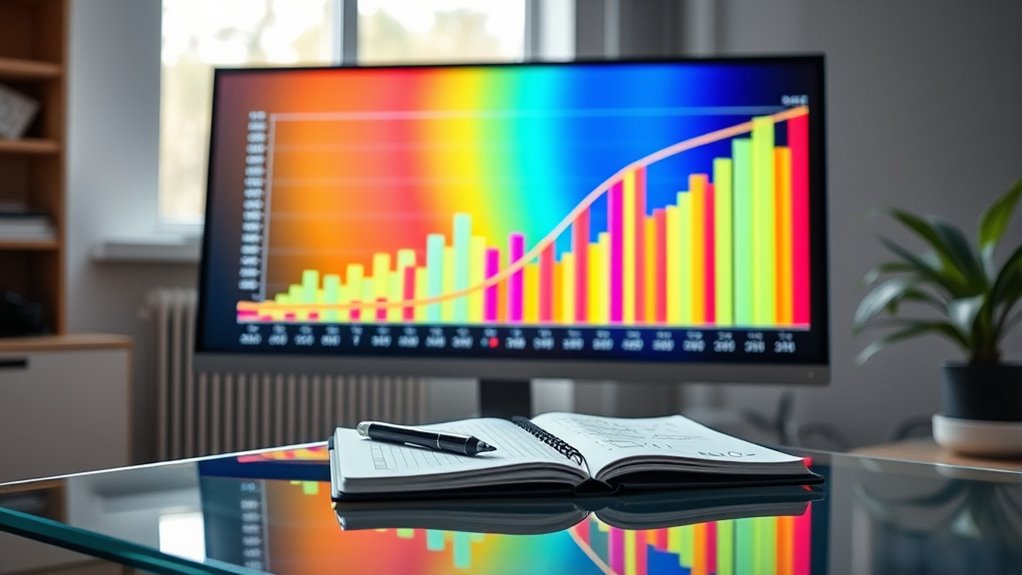
Visualizing data distributions with the mean allows you to see how the average fits within the overall pattern of your data. Using tools like histograms, you can add a vertical line to mark the mean, making it easy to assess whether data clusters around that value.
Box-and-whisker plots subtly include the mean by comparing it with quartiles, offering insights into data symmetry. Density and smooth histograms give a continuous view of distribution, with mean indicators enhancing interpretation.
In software like Python’s Seaborn or R’s ggplot2, adding mean lines is straightforward, helping you identify central tendencies quickly. Clear labels, contrasting colors, and appropriate plot types ensure your visualization accurately reflects the data’s spread and helps you communicate findings effectively.
Frequently Asked Questions
How Does the Mean Differ From Median and Mode?
Think of the mean, median, and mode as different navigators guiding you through data terrain. The mean is like an average traveler’s journey, summing all values and dividing.
The median is the median mountain’s peak, the middle value after sorting. The mode is the most popular stop, the most frequent value.
Each offers unique insights, with the mean sensitive to outliers, while the median and mode provide more robust perspectives.
Can the Mean Be Used for Categorical Data?
You might wonder if you can use the mean for categorical data. Generally, it’s not appropriate because categorical data, like nominal or ordinal types, lack meaningful numeric values or equal intervals.
Instead, focus on measures like the mode for nominal data or the median for ordinal data.
For certain ordinal scales, assigning numbers can help estimate the mean, but remember, these are just approximations and should be used cautiously.
What Are the Limitations of Using the Mean?
While the mean offers a straightforward way to understand data, it has its subtle shortcomings. You might find it overly influenced by extreme values, which can distort the true picture.
It’s less reliable with skewed or non-normal data, and not ideal for datasets with different units or scales.
For more robust insights, consider alternative measures like the median, especially when outliers or distribution issues are present.
How Do I Handle Missing Data When Calculating the Mean?
When handling missing data for calculating the mean, you can use mean imputation by replacing missing values with the average of available data. This method is simple and quick, especially for small missing data portions.
However, be aware it can bias variance estimates and reduce data variability. Always inspect your data first, validate your imputation, and document your approach for accurate and reliable analysis.
Is the Mean Affected by Data Distribution Shape?
Yes, the mean is affected by the data distribution shape. If your data is symmetric, the mean accurately reflects the average.
But in skewed distributions, the mean can be pulled toward outliers or extreme values, making it less representative of typical data points.
You’ll find the median often more reliable for skewed data, as it’s less sensitive to outliers and better captures the central tendency in such cases.
Conclusion
Now that you know how to calculate the mean, you can confidently analyze data sets and uncover insights. Remember, the mean offers a central value, but it’s not always the complete story—are you considering outliers or skewed data? By applying these steps carefully, you’ll improve your data interpretation skills. So, aren’t you ready to take your statistical understanding to the next level and make smarter decisions with your data?