To use SPSS for data analysis, first install the software and set up your license. Import your data files, like Excel or CSV, and organize the variables. Clean and prepare your data by checking for errors and missing values. Then, access Descriptive Statistics to explore your data or apply advanced techniques such as regression or multivariate tests for deeper insights. Keep exploring the steps to uncover powerful analysis tools with SPSS.
Key Takeaways
- Import your data files (Excel, CSV, text) into SPSS and save as .sav for easier analysis.
- Clean and validate data using SPSS tools to identify outliers and handle missing values.
- Conduct descriptive statistics via Analyze > Descriptive Statistics to summarize data features.
- Choose appropriate statistical procedures (e.g., t-tests, ANOVA, regression) based on your research questions.
- Interpret output results carefully, considering assumptions, significance levels, and data patterns for meaningful insights.
Installing and Setting Up SPSS
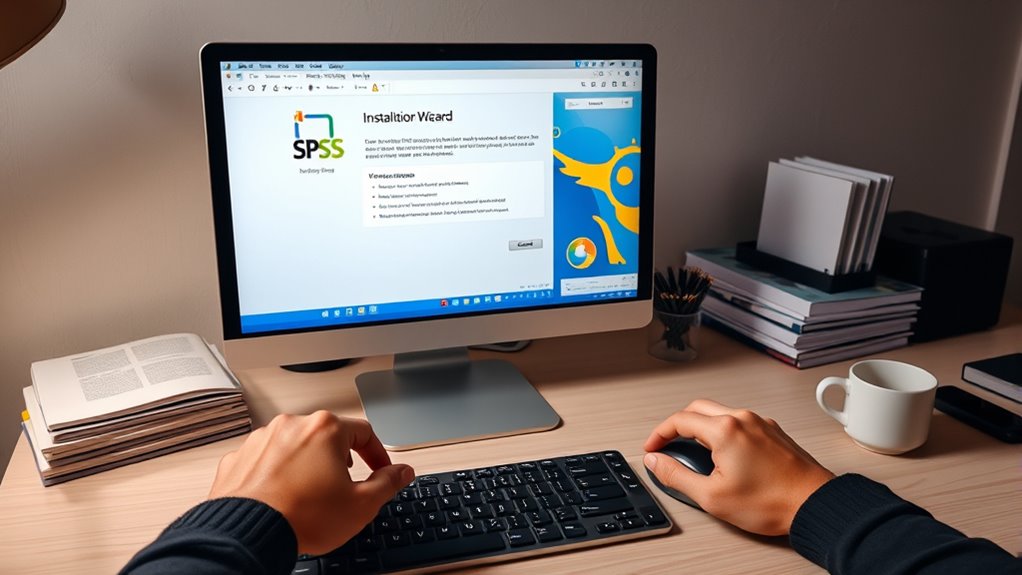
To begin using SPSS for data analysis, you first need to install and set up the software properly. Start by downloading SPSS from authorized sources like the IBM Marketplace or your institution’s library website. You’ll need to create or sign in with an IBM account to access the installer.
Make sure to select the correct version and download the appropriate installer file, such as SPSS_Statistics_win64.exe for Windows. Before installation, verify that your operating system is compatible with the software.
Run the installer and follow the on-screen prompts, accepting the license agreement and choosing the default or preferred installation directory. Once the installation completes, launch the License Wizard to activate SPSS by entering your license key, ensuring all components are properly installed and functioning.
Importing Your Data Into SPSS
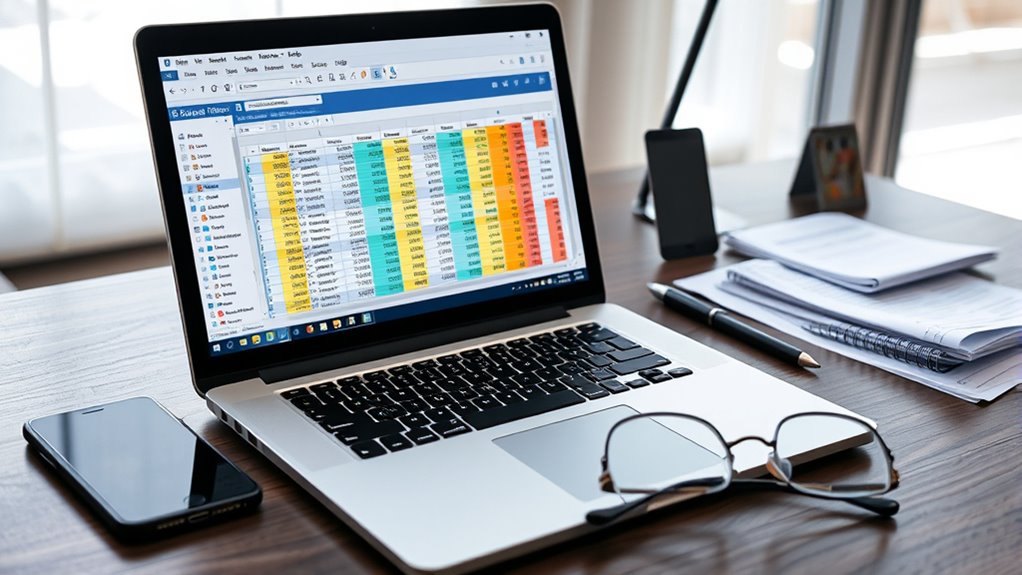
Importing your data into SPSS is a straightforward process that allows you to analyze information stored in various formats. To import Excel data, verify your spreadsheet is well-organized, with variable names in the first row and data below. Use “File” > “Import Data” to select and open your Excel file, then save it as an SPSS (.sav) file for easy access. For text or CSV files, similarly, choose “File” > “Import Data” and specify the correct delimiters and encoding. Make sure your files are organized properly, with clear variable names. Check for consistent data formatting to prevent errors. Additionally, understanding contrast ratio can help you evaluate the quality of your visual data representations within SPSS. Once imported, review your data in Data View, and save your work regularly. Proper data preparation guarantees a smooth transition into your analysis phase.
Managing and Preparing Data for Analysis
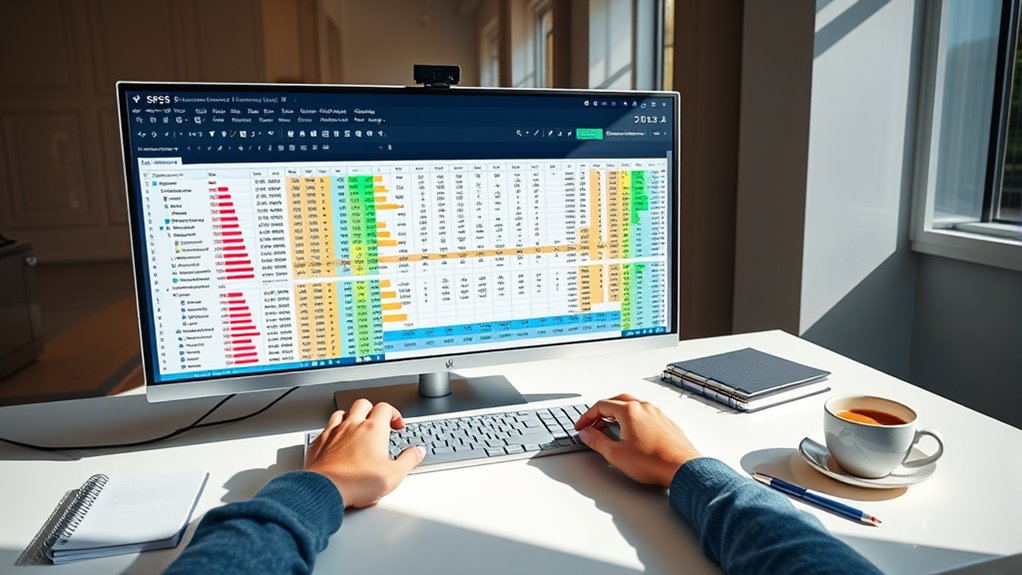
Effective data management and preparation are essential steps before diving into analysis, guaranteeing your dataset is accurate, consistent, and ready for meaningful insights. Start by validating your data using SPSS’s tools to identify invalid entries based on variable measure levels. Use automated checks to save time and reduce errors, and define variable types—categorical or continuous—to guide your validation process. Address suspicious cases or outliers that could skew results. Handle missing data by examining patterns and evaluating data completeness. Utilize SPSS’s automated data preparation features to identify and fix issues efficiently. For better model accuracy, consider binning your variables using SPSS’s optimal binning procedures, choosing among unsupervised, supervised, or hybrid methods as appropriate. Additionally, understanding the importance of data quality can significantly enhance your analysis outcomes. Proper management ensures your data is clean, consistent, and analysis-ready. Incorporating powerful persuasive words can further improve your data presentation and interpretation, making your findings more compelling and actionable.
Conducting Descriptive Statistics
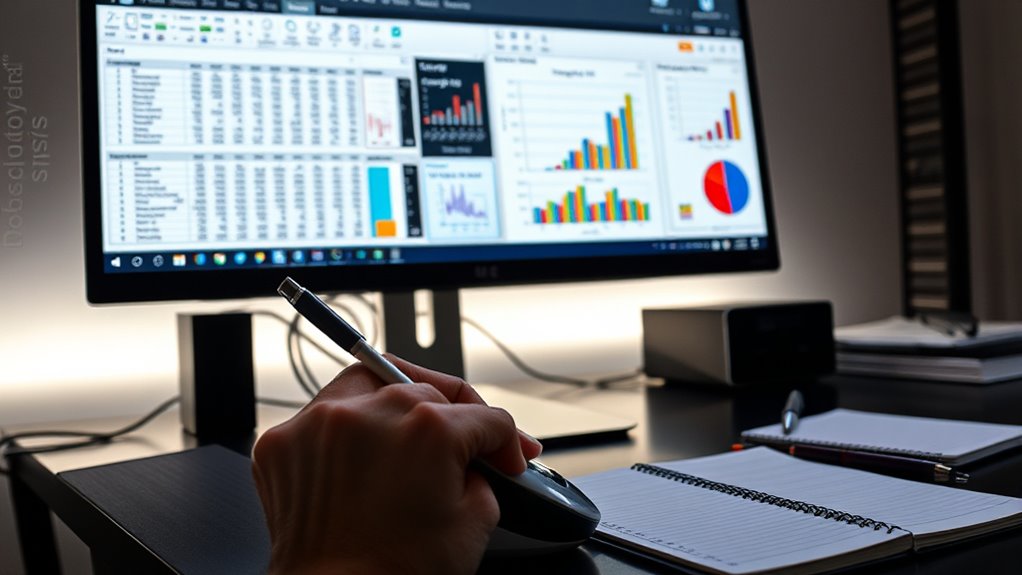
Conducting descriptive statistics provides a clear overview of your dataset’s key features, helping you understand data distribution, central tendency, and variability.
In SPSS, you access this through Analyze > Descriptive Statistics > Descriptives. Select the variables you want to analyze, ensuring they’re properly labeled and formatted.
The output includes measures like mean, median, mode, standard deviation, and variance, which reveal how data points are spread and centered. These statistics help identify outliers or patterns and assess data quality.
Remember, descriptive stats are mainly for numeric variables; categorical data requires frequency analysis. Use the output tables for reporting, and consider visual aids for clarity.
Conducting these analyses gives you essential insights to guide further data exploration and interpretation.
Performing Advanced Statistical Procedures
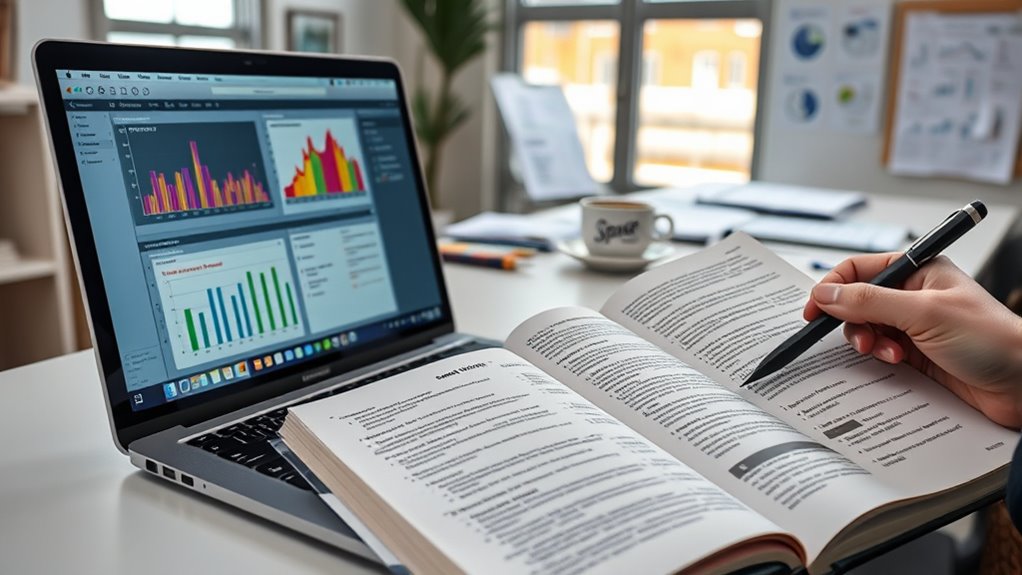
Performing advanced statistical procedures in SPSS allows you to analyze complex data structures and answer sophisticated research questions. You can apply linear models for normally distributed variables or use logistic and probit models for binary outcomes. Maximize space and organization techniques can enhance data management and clarity within your analysis. Loglinear models help analyze count data, while nonstandard regression options like ordinal, Poisson, or negative binomial regression address specific data types. Mixed models enable predictions with nonlinear outcomes. Multivariate techniques such as discriminant analysis, MANOVA, and factor analysis help classify data, reduce dimensions, and explore underlying factors. For correlated data, repeated measures ANOVA, multilevel models, and split-plot designs account for variability at different levels. These advanced procedures expand your analytical capabilities, providing deeper insights into complex datasets.
Visualizing and Interpreting Results
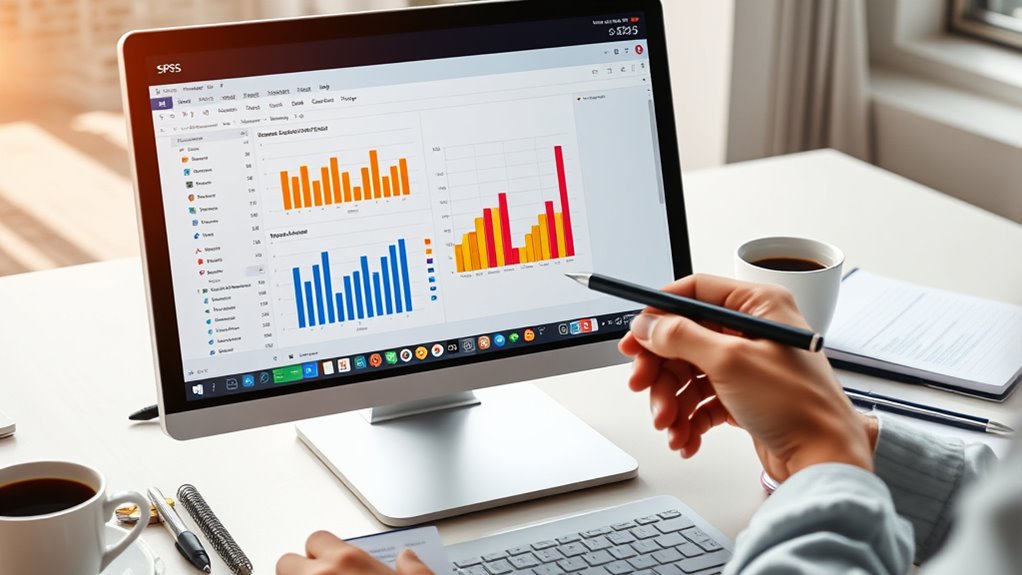
Visualizing and interpreting results in SPSS helps you make sense of complex data by turning numbers into meaningful insights. You can create various charts like bar, pie, and line plots to display raw data, central tendencies, and patterns. Choosing the right graph depends on your data and research questions, and SPSS allows customization to improve clarity and readability. Utilizing essential oils for specific health concerns can further enhance your understanding of data related to natural remedies. Visualizations help identify trends, compare groups, and detect outliers or missing data, offering a clearer picture than raw numbers alone. Managing data through filtering, cleaning, and transformation ensures accurate visuals. Understanding operating hours is crucial for planning visits to entertainment parks and can be visualized effectively to compare seasonal or daily schedules. Advanced techniques include visualizing interactions in multifactorial designs and customizing graphs further.
Remember to keep your visualizations simple, audience-focused, and refined through feedback for maximum impact.
Frequently Asked Questions
How Do I Troubleshoot Common Errors During SPSS Installation?
When troubleshooting common SPSS installation errors, you should first check for permission issues by granting full control to the installation folder.
Confirm there’s enough disk space and verify that files aren’t corrupted.
Review error messages carefully, and try reinstalling if needed.
Also, verify your system meets all requirements, update your OS, and consider contacting support if problems persist.
These steps help resolve most installation hurdles efficiently.
Can SPSS Handle Large Datasets Efficiently?
Yes, SPSS can handle large datasets efficiently.
You can optimize performance by leveraging database integration, pushing operations closer to the data source, and using data reduction techniques like sampling or aggregation.
Guarantee your hardware has sufficient RAM and processing power, and use 64-bit versions for better memory management.
Regularly monitor system performance, preprocess data beforehand, and consider parallel processing to speed up analysis of big data.
What Are Best Practices for Cleaning Data in SPSS?
Cleaning data in SPSS is like tidying up a cluttered room—you need a plan. Start by backing up your data and assigning unique IDs.
Inspect for errors, missing values, and outliers. Use SPSS tools to correct errors, impute missing data, and standardize formats.
Document every change for transparency. Finally, verify your cleaned data, update backups, and guarantee everything is consistent before diving into analysis.
How Do I Customize SPSS Output for Reporting?
When customizing SPSS output for reporting, you control how data appears by adjusting fonts, colors, and table layouts. Access the “Options” menu to change output formats or styles, ensuring your report aligns with guidelines like APA.
You can also modify decimal points, clean charts, and save templates for consistency. Export your final output as PDF, Excel, or CSV, and remove unnecessary elements to create clear, professional reports.
Is There a Way to Automate Repetitive Analyses in SPSS?
Ever wondered if you can save time with your analyses? Yes, you can automate repetitive tasks in SPSS using syntax. By scripting your procedures, you eliminate manual effort, reduce errors, and speed up your workflow.
You can schedule analyses, handle various datasets seamlessly, and even integrate with other tools. With some training, you’ll master automation, making your work more efficient and reliable—changing how you approach data analysis forever.
Conclusion
Think of using SPSS like steering through a well-organized toolbox. When you know how to import, prepare, and analyze your data, you’re assembling the perfect toolkit to uncover insights. Just like a mechanic expertly uses each tool to fix a car, you’ll confidently interpret results and visualize data. With practice, SPSS becomes your trusted partner, turning raw numbers into clear stories—helping you open the full potential of your data, one step at a time.