A p-value helps you understand whether your experiment’s results are likely due to chance or indicate a real effect. It shows the probability of observing your data if the null hypothesis is true. A low p-value (typically below 0.05) suggests your results are statistically significant, meaning they are unlikely to happen by chance alone. To get a clearer picture and avoid common mistakes, explore how p-values work and what they really mean.
Key Takeaways
- A p-value shows how likely your data would occur if there was no real effect or difference.
- A small p-value (typically ≤ 0.05) suggests the results are unlikely due to chance alone.
- It helps decide whether to reject the null hypothesis, not how important or big the effect is.
- A high p-value indicates the data is consistent with the null hypothesis; a low p-value suggests evidence against it.
- P-values do not prove a hypothesis is true or show the probability that it is true.
What Is a P-Value and Why Does It Matter?
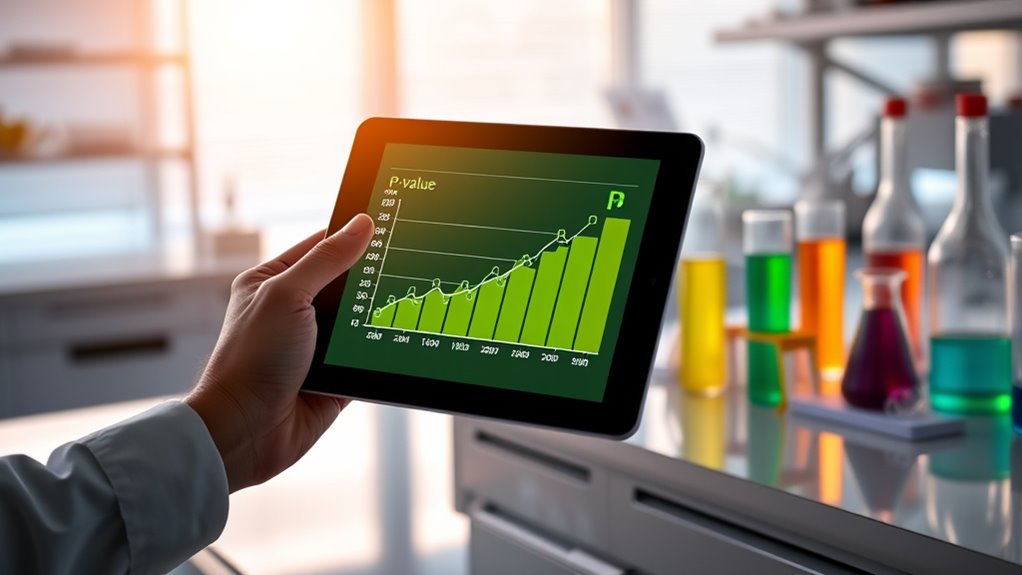
Have you ever wondered how researchers determine if their results are meaningful or just due to chance? That’s where a p-value comes in. It measures the probability of getting your observed data if the null hypothesis is true.
A p-value indicates the likelihood of obtaining your results if the null hypothesis is true.
A low p-value suggests your results are unlikely to be caused by chance alone, providing evidence against the null hypothesis. Typically, a p-value of 0.05 or less is considered statistically significant, meaning the findings are unlikely to be random. Recognizing the statistical significance of findings helps in making informed decisions based on data.
P-values help you decide whether to reject the null hypothesis, guiding research conclusions. However, they don’t tell you about the practical importance of the results or the size of the effect.
Used correctly, p-values are a key tool in understanding the significance of your data. Dream symbols can sometimes be used metaphorically to represent the significance or underlying meaning of statistical results in research.
How Are P-Values Calculated and Used?
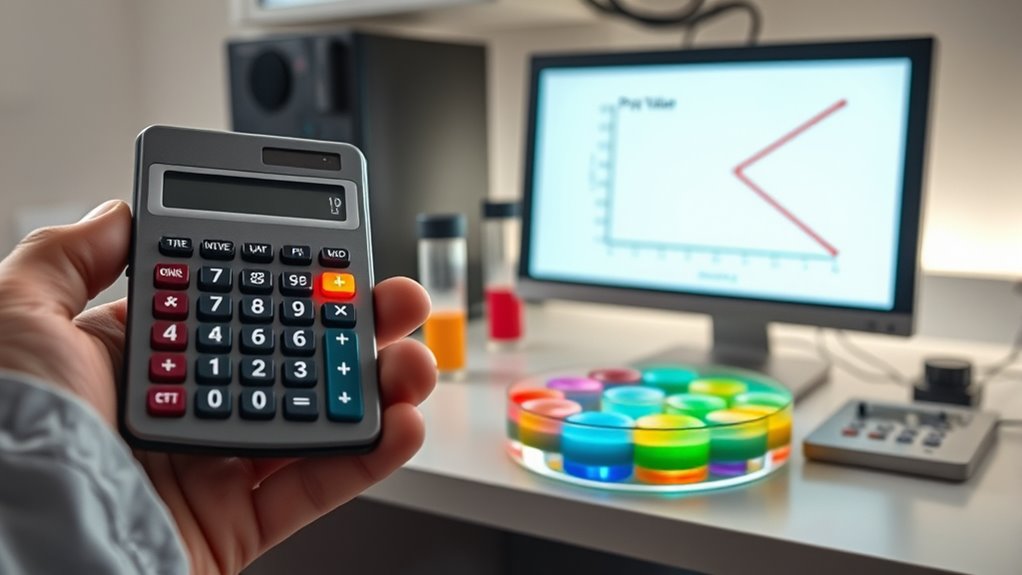
Calculating p-values involves determining the probability of observing data as extreme or more extreme than what you actually found, assuming the null hypothesis is true. To do this, you first calculate a test statistic, like a t-value, using formulas that incorporate your sample mean, standard deviation, and sample size. Once you have the statistic, you look at the relevant probability distribution—such as the normal or t-distribution—and find the area under the curve beyond your observed value. This area represents the p-value. Typically, software or tables help with this calculation, especially for complex integrals. The p-value then indicates how likely your data is if the null hypothesis is holds, guiding whether to reject or not reject H0. Understanding statistical distributions is crucial for correctly interpreting your results and making informed decisions based on p-values. To better interpret these distributions, familiarity with probability theory can be very helpful.
Interpreting P-Values in Research and Testing
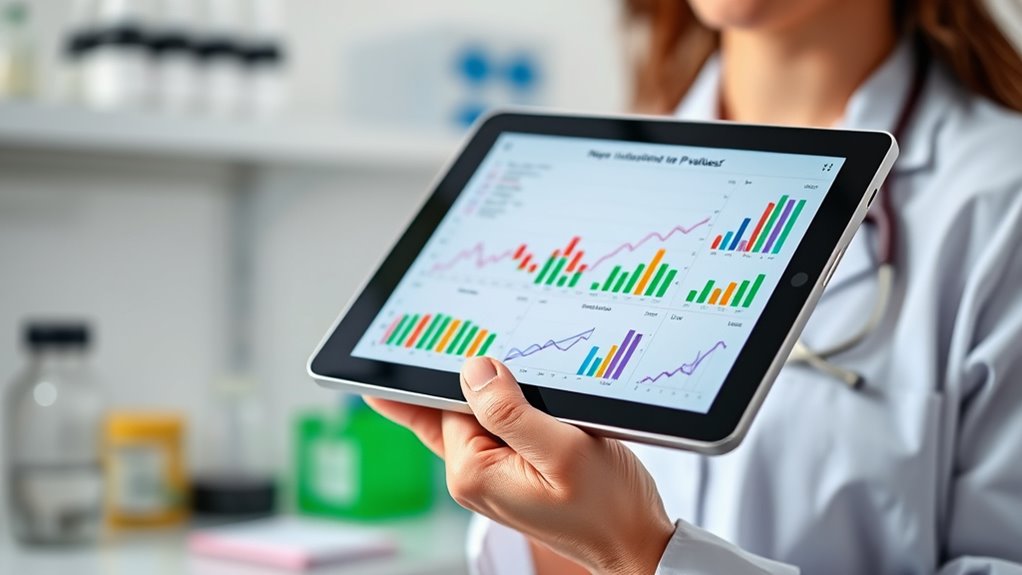
Interpreting p-values correctly is essential for understanding research results and making informed conclusions. A p-value shows how strong the evidence is against the null hypothesis, but it’s not a simple yes-or-no answer. Instead, think of it as a range of evidence, with smaller values indicating stronger evidence of an effect. Recognizing the financial implications of research findings can also influence how results are applied in real-world scenarios. Understanding the key traits of successful researchers can also help interpret statistical results more effectively. Comparing the p-value to your chosen alpha level (like 0.05) helps decide if the result is statistically significant. Remember, a low p-value doesn’t prove the alternative hypothesis true; it just suggests the observed data is unlikely if the null is true.
Context matters—sample size, test type, and field-specific standards all influence interpretation. Avoid assuming p-values provide definitive proof; they’re just one piece of the research puzzle.
Common Mistakes and Misunderstandings About P-Values
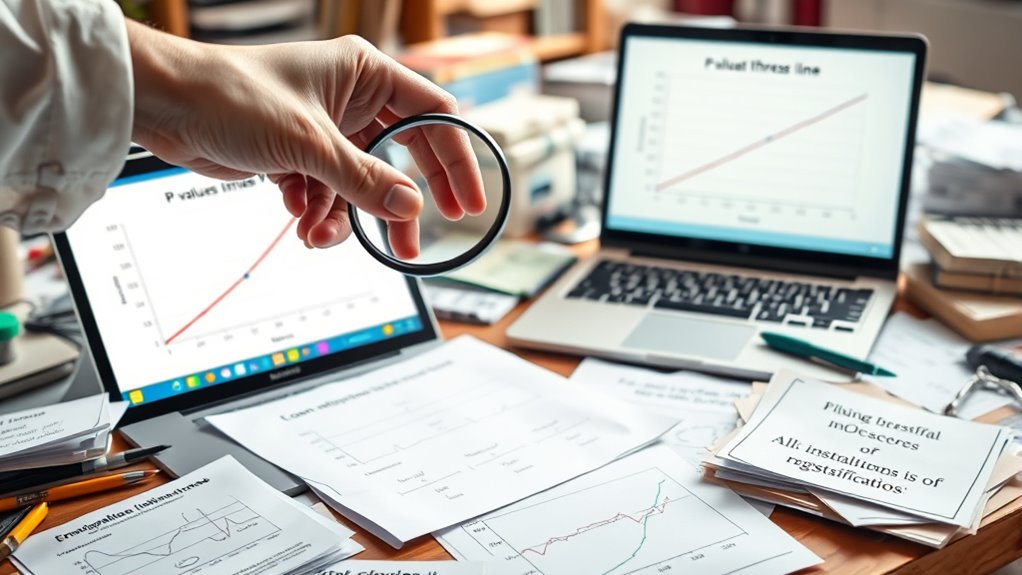
Misunderstandings about what p-values actually tell us are common and can lead to flawed conclusions. Many believe a p-value shows the probability that the null hypothesis is true, but it only indicates how unlikely the observed data would be if the null were true. A new sentence with independent data and the rest of the sentence. You might think a low p-value means a high chance the null is false, but that’s incorrect. It doesn’t measure effect size or importance, only data compatibility with the null. Some assume statistical significance equals practical significance, which isn’t always true. Others compare p-values across studies without considering context or multiple testing, increasing the risk of false positives. Recognizing these misconceptions helps you interpret p-values more accurately, avoiding pitfalls that could mislead your research conclusions. Additionally, understanding statistical power can aid in designing better studies and interpreting results more reliably.
Practical Examples of P-Values in Action
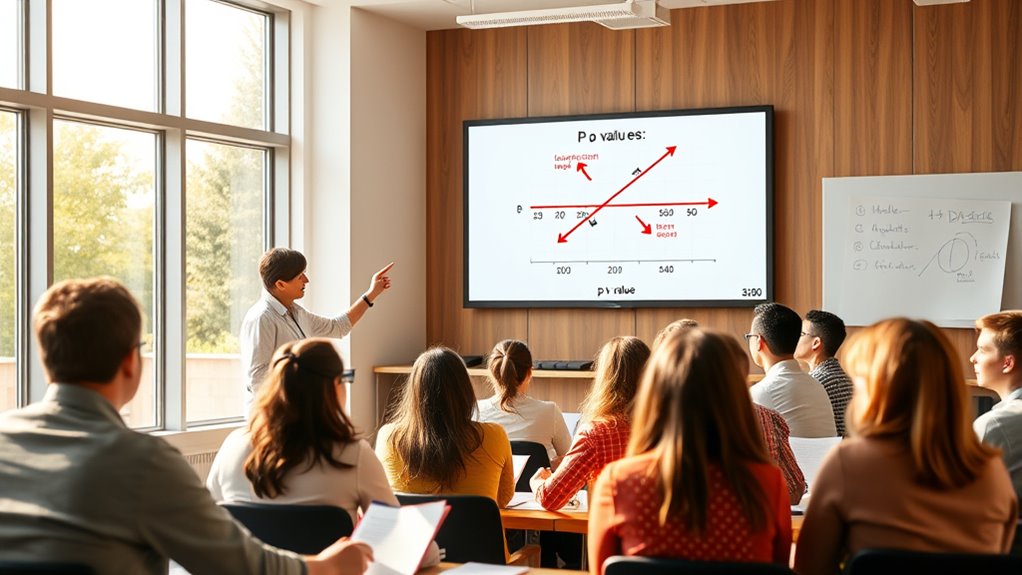
P-values play a pivotal role in various real-world decision-making scenarios, helping researchers and professionals determine whether observed effects are likely due to chance or represent meaningful differences.
For example, in medical research, a low p-value (below 0.05) suggests a new drug may truly improve patient outcomes, guiding approval decisions.
In investment analysis, a small p-value indicates that a portfolio’s performance differs considerably from the benchmark, supporting strategies to adjust or maintain holdings.
Environmental scientists use p-values to identify whether pollution levels substantially impact ecosystems, informing policy actions.
Automotive engineers rely on p-values to verify if vehicles meet fuel efficiency standards beyond random variation.
Additionally, dog-related products like specialized dog beds or nutritious meals can be selected based on evidence of their benefits, highlighting the importance of data-driven decisions. Understanding statistical significance helps ensure that conclusions drawn from data are reliable and not just due to chance.
These examples show how p-values help assess evidence, guiding decisions across fields by distinguishing real effects from random noise.
Frequently Asked Questions
Can a P-Value Prove a Hypothesis Is True?
You ask if a p-value can prove a hypothesis is true. The answer is no.
P-values only tell you the likelihood of your data assuming the null hypothesis is true; they don’t confirm the hypothesis itself.
A low p-value suggests evidence against the null, but it doesn’t prove the hypothesis is correct.
You need other evidence and methods to truly support or confirm a hypothesis.
How Does Sample Size Affect P-Value Interpretation?
Imagine doubling your sample size; your P-value drops from 0.06 to 0.01, making results seem more significant. Larger samples increase statistical power, helping you detect real effects more easily.
They also make P-values more sensitive, often lowering them, even for tiny differences. But beware—big samples can show statistically significant results for trivial effects, so always interpret P-values within the study’s context and effect size for meaningful conclusions.
Is a Lower P-Value Always Better?
You might think a lower p-value is always better, but that’s not necessarily true. While lower p-values suggest stronger evidence against the null hypothesis, they don’t measure the importance or practical significance of your findings.
A very low p-value can occur even with a tiny effect that isn’t meaningful in real-world terms. Focus on the context and effect size, not just the p-value, to make better interpretations.
What Is the Difference Between P-Value and Effect Size?
Did you know that a p-value of 0.01 means there’s just a 1% chance your results happened by luck?
The difference between p-value and effect size is that p-value shows whether the result is statistically significant, while effect size measures how meaningful or large that result really is.
You need both to understand if your findings aren’t only reliable but also practically important.
Are P-Values Useful in All Types of Research Studies?
You ask if P-values are useful in all research studies. They’re mainly helpful in quantitative research where numerical data allows for statistical testing.
However, in qualitative studies, P-values are less applicable because they don’t measure non-numerical data. Even in quantitative research, P-values shouldn’t be the sole focus; consider effect sizes and practical significance to fully understand your findings.
Always interpret P-values within the specific context of your study.
Conclusion
Now that you understand p-values, you hold the power to unlock the secrets of research like a detective with a magnifying glass. Don’t let misunderstandings turn you into a confused maze runner — instead, confidently navigate the data jungle, spotting truths and myths with laser precision. With this knowledge, you can cut through the fog of statistics and see the world of science clearly, as if you’ve just discovered the hidden door to ultimate understanding.