To avoid common statistical mistakes, don’t forget to correct for multiple comparisons so your results aren’t false positives. Be cautious when interpreting P-values; they don’t prove causation or practical significance. Using small sample sizes can lead to unreliable conclusions. Remember that indirect comparisons are misleading—you need direct tests. Also, never treat correlations as causations, as other factors may be involved. Stay aware of these pitfalls to improve your analysis and uncover more valuable insights.
Key Takeaways
- Correct for multiple comparisons to prevent false positives and ensure valid significance testing.
- Interpret P-values accurately as measures of data probability, not proof of causality or effect.
- Use adequate sample sizes to improve estimate reliability, statistical power, and generalizability.
- Make direct comparisons between groups rather than relying on separate significance tests.
- Avoid assuming causation from correlation; use proper experimental designs to establish cause-effect relationships.
Failing to Correct for Multiple Comparisons
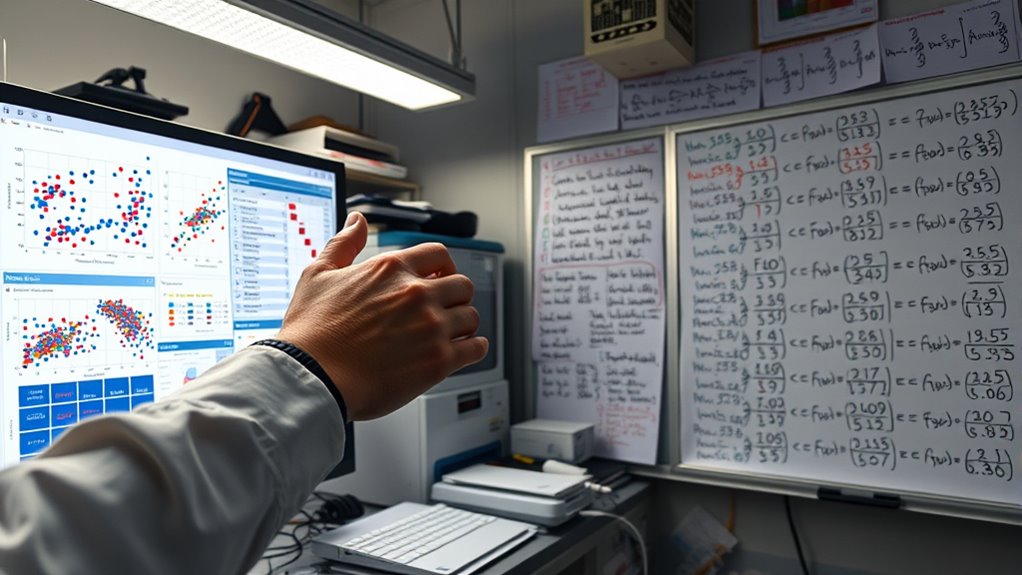
Failing to correct for multiple comparisons can lead you to draw incorrect conclusions from your data. When you test many hypotheses simultaneously, the chance of false positives (Type I errors) increases, making it seem like your results are significant when they’re not. Breakfast delivery options such as smoothies, omelets, and artisanal items are often tested across different groups, which can inflate the likelihood of identifying spurious effects if not properly corrected. Without proper correction methods, you risk overestimating the effects you’re studying. While some correction techniques, like the Bonferroni method or False Discovery Rate control, reduce false positives, they can also lower your study’s power. Ignoring experiment design and not reporting all results transparently can further skew your findings. To maintain accuracy, plan your hypotheses carefully, apply suitable correction methods, and clearly report your approach. Implementing statistical corrections helps ensure that your results are reliable. This way, your conclusions will genuinely reflect your data’s true significance.
Misinterpreting P-Values
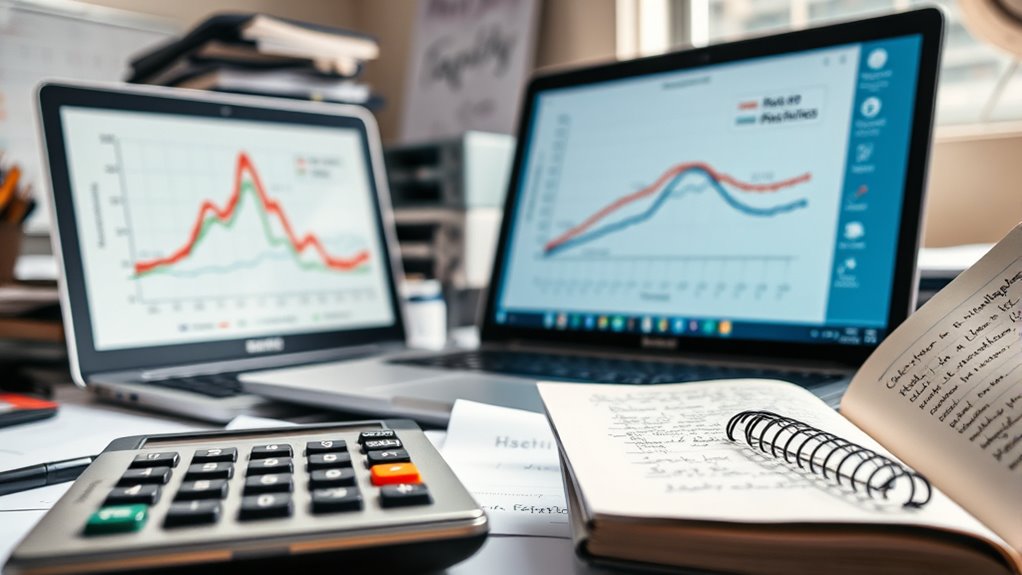
Misinterpreting P-values is a common mistake that can lead to false conclusions about research findings. Many people mistakenly believe that a low P-value shows strong evidence for the alternative hypothesis or that it indicates the null hypothesis is unlikely.
In reality, a P-value measures the probability of observing data as extreme or more extreme if the null hypothesis is true, not the probability that the null hypothesis itself is false. This misunderstanding often results in overconfidence in statistically significant results and ignores the importance of practical significance. The complexity of data privacy challenges further emphasizes the need for careful interpretation of statistical results to avoid misleading conclusions.
Even experts can misunderstand P-values, as surveys reveal that only about 62% interpret them correctly. Remember, a P-value is just one piece of the puzzle and shouldn’t be the sole basis for drawing conclusions. Additionally, the misuse of P-values can be compounded by issues such as cybersecurity vulnerabilities during system outages, which highlight the importance of comprehensive data verification and validation processes.
Using Small Sample Sizes
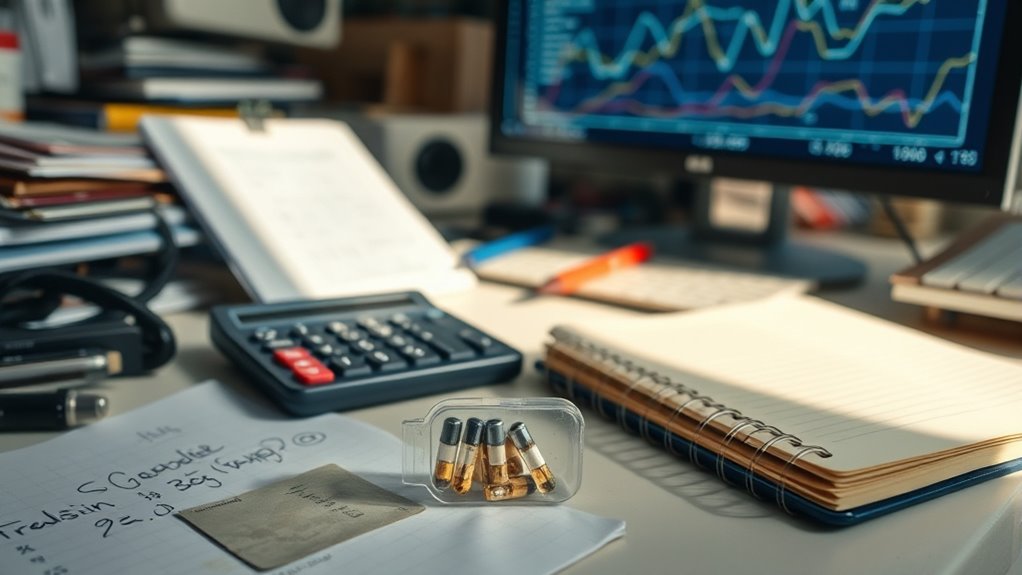
Using small sample sizes in research can substantially undermine the validity and reliability of your findings. When your sample is too small, your estimates of population parameters become less trustworthy, increasing the chance of incorrect conclusions.
Small samples lead to wider confidence intervals and a higher margin of error, making your results less precise. They also reduce the statistical power, lowering the likelihood of detecting true effects and increasing the risk of Type I and Type II errors.
Additionally, models based on limited data struggle to meet assumptions like normality and equal variance, which can distort your analysis. Overall, small samples compromise the generalizability of your findings and make them more susceptible to chance fluctuations, reducing their robustness and reproducibility.
Planning for adequate sample sizes is essential for valid, meaningful research.
Making Indirect Comparisons
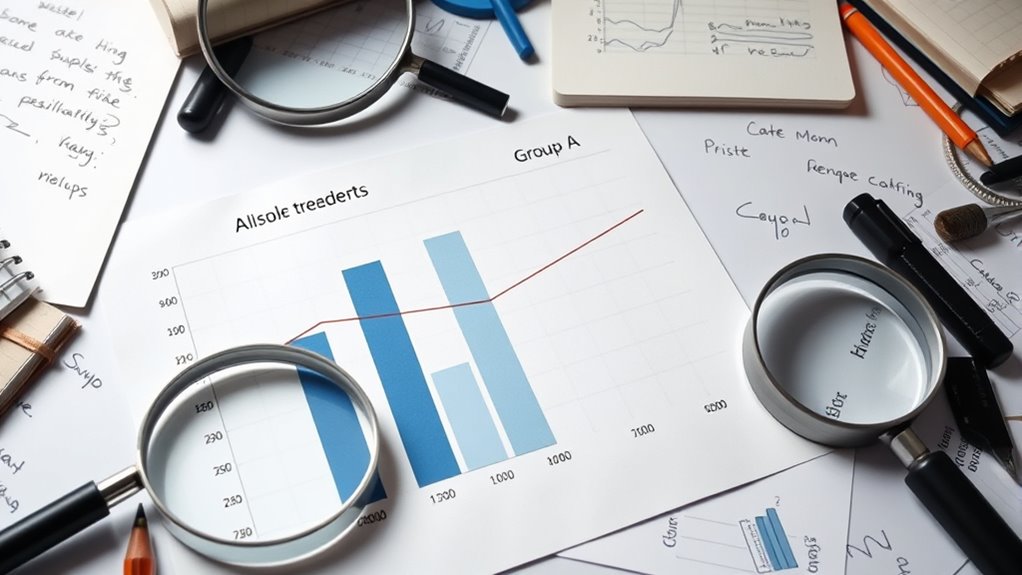
Making indirect comparisons involves drawing conclusions about the relationship between two groups based on separate analyses rather than direct statistical tests. This approach can lead to incorrect inferences about effect sizes and differences. Additionally, understanding the importance of zodiac sign compatibility can help contextualize differences in personal or cultural preferences, but it should not substitute for proper statistical comparison methods. For example, finding a significant effect in one group and not in another doesn’t mean the groups differ; p-values aren’t transitive. Relying on individual tests instead of direct comparisons like t-tests or ANOVA increases the risk of bias and misinterpretation. It’s essential to perform direct statistical comparisons to accurately assess group differences and avoid false conclusions. Using proper methods, such as pairwise tests or post-hoc analyses, ensures valid, reliable results. Recognizing sample size and variability is crucial for correct interpretation of statistical outcomes. Avoiding indirect comparisons helps prevent misconceptions and improves the integrity of your findings.
Treating Correlations as Causations
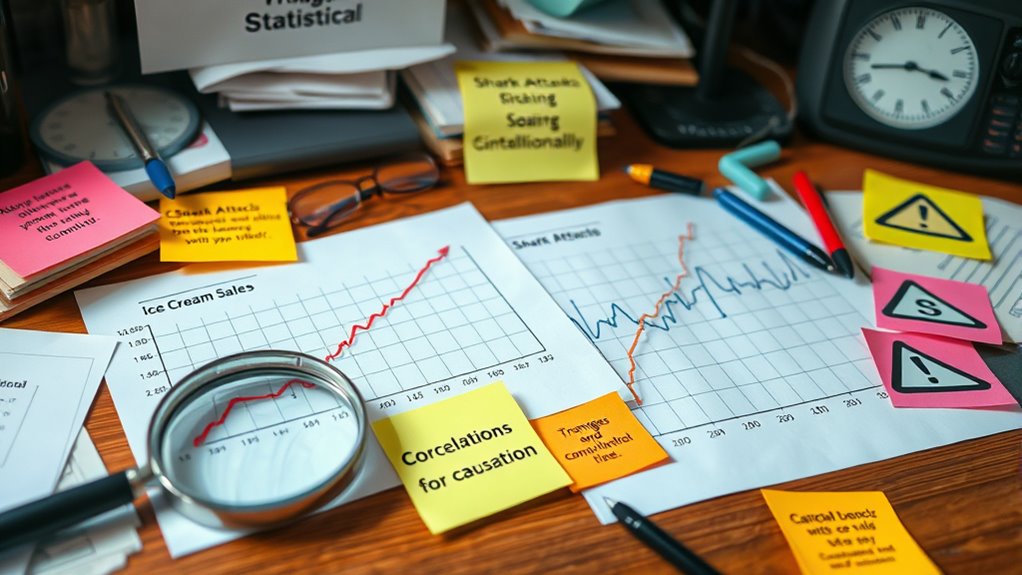
It’s common to see people assume that a correlation between two variables indicates a direct causal relationship, but this is a dangerous oversimplification. Just because two things change together doesn’t mean one causes the other.
For example, ice cream sales and heatwaves are correlated, but eating ice cream doesn’t cause hot weather. Relying on correlation alone can lead you to false conclusions and misguided policies.
Correlation coefficients measure the strength and direction of relationships but don’t prove causation. Many factors, like confounding variables, influence both variables, making it risky to jump to causality. Understanding statistical concepts can help in recognizing patterns and connections without assuming causality.
To avoid mistakes, use proper experimental designs, control groups, and long-term data to establish true cause-and-effect relationships, not just statistical associations. Additionally, understanding textile line concepts can help in recognizing patterns and connections without assuming causality.
Violating Assumptions of Statistical Tests
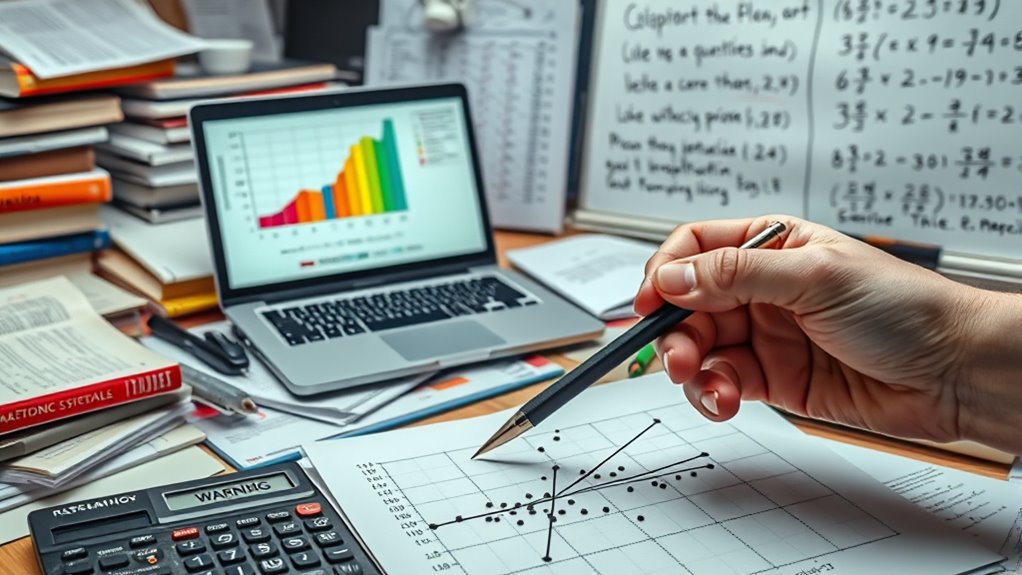
Assumptions behind statistical tests are often overlooked, but failing to meet them can seriously compromise your results. If your data aren’t normally distributed, tests like the t-test may produce inaccurate p-values, leading to false conclusions. For example, spoiled lemon juice often shows discoloration and separation, which may indicate non-normal distribution of spoilage signs. Using appropriate self watering plant pots can help maintain consistent moisture levels, reducing variability that might affect data normality in experiments. Unequal variances across groups can distort results unless you use alternatives like the Welch test. Dependence among data points violates independence assumptions, risking biased outcomes. Outliers or non-random sampling can distort estimates and affect the validity of your tests. To avoid these issues, visually inspect your data with histograms or Q-Q plots, and perform tests such as Shapiro-Wilk or Levene’s. If assumptions are violated, consider nonparametric methods, data transformations, or robust models. Properly checking and addressing these assumptions ensures your results are reliable and accurate.
Frequently Asked Questions
How Do I Determine the Appropriate Correction Method for Multiple Tests?
When choosing a correction method, you should consider your data type, the number of tests, and how tests relate.
If tests are independent, Šidák or Bonferroni might work well.
If tests are dependent, consider the Holm-Bonferroni or FDR-based methods like Benjamini-Hochberg.
Also, think about your research goals and software tools, as these factors influence which correction balances false positives and statistical power best.
What Are Practical Ways to Interpret P-Values Correctly?
Imagine P-values as tiny detectives with magnifying glasses, peering at data clues.
To interpret them correctly, don’t treat them as proof or fortune-tellers.
Remember, a low P-value hints at evidence against the null hypothesis, but it doesn’t confirm anything for sure.
Context matters.
Use them alongside study design, effect size, and practical significance.
Keep your detective work grounded—don’t jump to conclusions based solely on a number.
How Can I Calculate the Right Sample Size for My Study?
To calculate the right sample size, first define your study’s main outcomes and hypotheses.
Use appropriate formulas based on your design and desired confidence level and power.
Leverage reliable software, but double-check the results manually.
Consider factors like margin of error, population size, and variability.
Always document your assumptions, and consult a statistician if you’re unsure.
Proper planning guarantees your study yields accurate, meaningful results.
When Is It Valid to Make Indirect Comparisons Between Groups?
You can make indirect comparisons valid when the studies involved are sufficiently similar in patient populations, trial designs, and conditions.
Confirm the assumptions of similarity and homogeneity are met, and use appropriate statistical models like random effects to account for heterogeneity.
Always evaluate the consistency between direct and indirect evidence, and supplement your analysis with qualitative assessments to strengthen your conclusions.
How Do I Check if My Data Meet the Assumptions of Statistical Tests?
You wonder if your data meet the assumptions of statistical tests, but how can you be certain? First, check normality with skewness, kurtosis, or the Shapiro-Wilk test.
Then, verify homogeneity of variances using Levene’s or Bartlett’s test.
Finally, guarantee independence and linearity through scatter plots or residual analysis.
These steps reveal if your data are ready or if adjustments are needed before proceeding confidently.
Conclusion
Remember, avoiding these common mistakes can save your research from misleading conclusions. For example, failing to correct for multiple comparisons can inflate false positives by up to 50%, making your results unreliable. Always interpret p-values carefully, use adequate sample sizes, and recognize the difference between correlation and causation. By staying vigilant about assumptions and comparisons, you’ll ensure your analysis is robust and trustworthy, helping you draw meaningful and accurate insights from your data.