Bayesian statistics is a method for updating your beliefs based on new evidence. You start with a prior idea or belief about a situation, then gather data and apply Bayes’ theorem to revise this into a more accurate posterior belief. This process allows you to incorporate previous knowledge and quantify uncertainty effectively. If you keep exploring, you’ll discover how these principles power decision-making and innovative data analysis techniques.
Key Takeaways
- Bayesian statistics updates beliefs using new data through Bayes’ theorem, combining prior knowledge with observed evidence.
- It begins with a prior distribution reflecting existing beliefs, which is revised into a posterior after data analysis.
- The approach quantifies uncertainty and supports continuous learning and adaptation over time.
- Computational techniques like MCMC help estimate complex posterior distributions when analytical solutions are difficult.
- It is widely used across fields like healthcare, finance, and AI for decision-making, risk assessment, and hypothesis testing.
Understanding the Core Principles of Bayesian Thinking
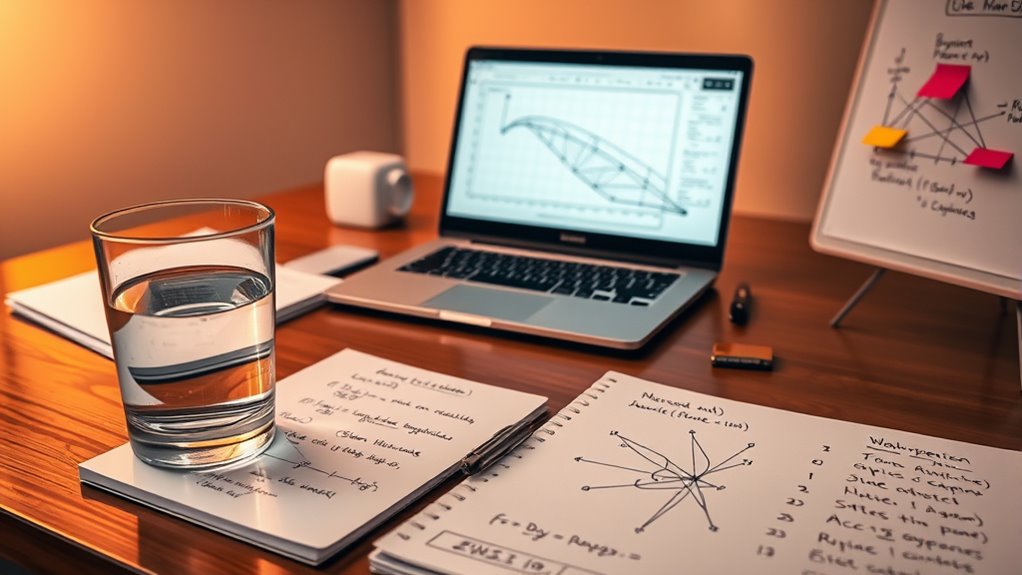
To truly understand Bayesian thinking, you need to recognize that it centers on updating your beliefs with new evidence. You start with a subjective belief, called a prior, about a parameter or event. When new data arrives, you use Bayes’ theorem to combine this prior with the likelihood of the data, resulting in a revised belief called the posterior. A solid understanding of probability concepts enhances the application of Bayesian methods. This process is grounded in conditional probability, which calculates the chance of an event given another. Unlike frequentist methods, Bayesian approaches systematically incorporate prior knowledge, making your inferences more coherent. The entire process creates a self-consistent framework that adapts as new evidence emerges. By quantifying degrees of belief, Bayesian thinking provides a flexible, iterative way to refine your understanding based on the most current information. Additionally, understanding the role of prior distributions is crucial for applying Bayesian analysis effectively.
How Bayesian Inference Updates Beliefs With New Data
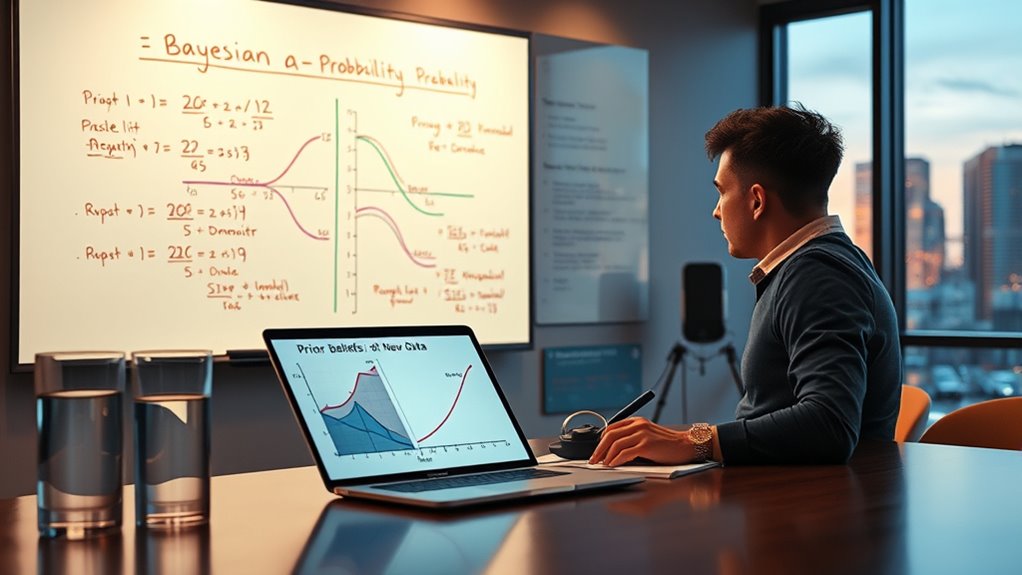
Bayesian inference updates beliefs by systematically combining prior knowledge with new data through Bayes’ theorem. When you receive fresh evidence, you integrate it with your existing understanding by calculating the likelihood of the new data under different hypotheses. Auditory processing techniques help inform your expectations about skin improvement, enhancing the process of updating beliefs. This process results in a revised belief called the posterior distribution. As you gather more data, the posterior becomes your new prior, allowing for continuous refinement of your model. This iterative process helps you adapt your beliefs dynamically, reflecting emerging information.
Bayesian inference also quantifies uncertainty, so you know how confident you’re in your updated beliefs. It’s especially powerful in complex models and small sample situations, where traditional methods might struggle.
Ultimately, this approach enables you to learn from data consistently and make more informed decisions.
The Role of Prior Distributions in Bayesian Analysis
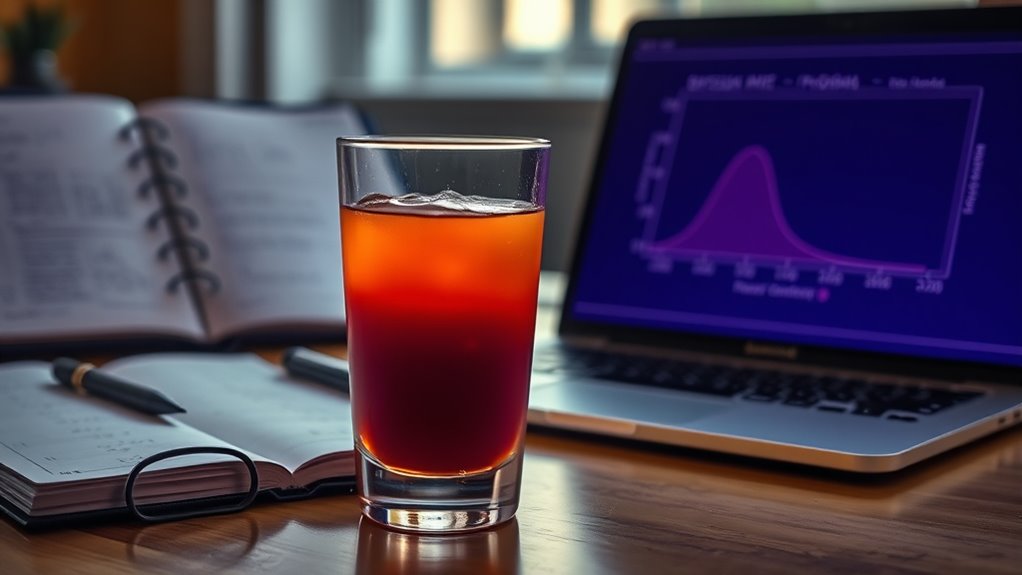
Prior distributions serve as the foundation for Bayesian analysis by representing your existing knowledge or beliefs about parameters before examining new data. They combine with the likelihood to produce the posterior, which guides your inference. Incorporating prior distributions aligned with sustainable practices can enhance the robustness of your analysis. Choosing the right prior is essential; it should reflect what you already know or your level of skepticism. Priors can be informative, incorporating substantial prior knowledge, or non-informative, aiming to minimize influence when little is known. Proper priors have a well-defined probability distribution, while improper priors do not. The impact of the prior decreases as your sample size grows, but in small samples, it can heavily influence results. Understanding the role of priors in different contexts helps you select the most appropriate prior for your analysis. Sensitivity analysis helps you understand how different priors affect your conclusions, ensuring your inferences are robust.
Applying Bayes’ Theorem to Real-World Problems
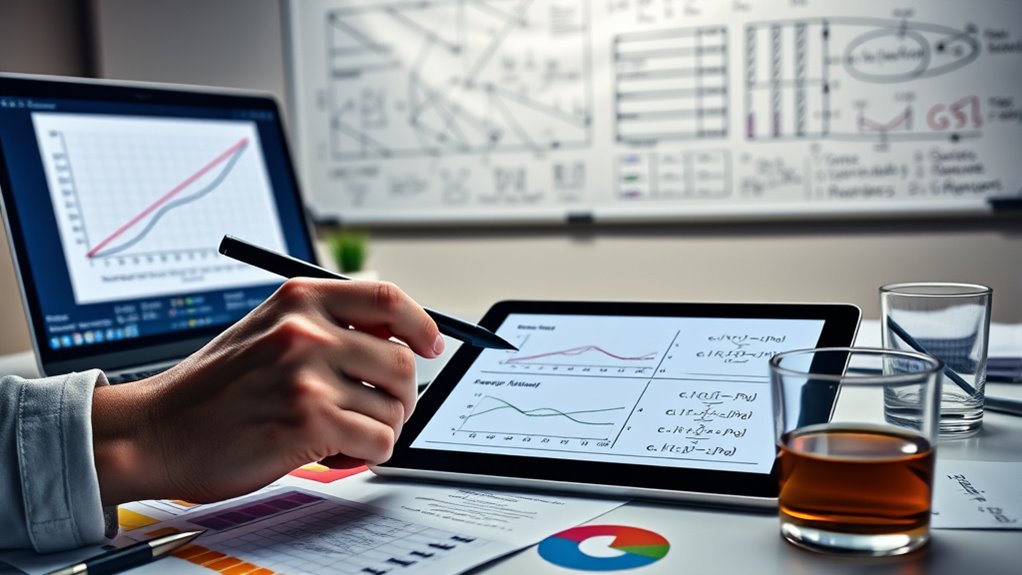
Applying Bayes’ Theorem to real-world problems allows you to update your beliefs and make informed decisions as new evidence emerges. In healthcare, it helps interpret medical test results more accurately by combining symptoms and patient history. Additionally, understanding conflict resolution skills can improve decision-making in high-stakes situations by evaluating the reliability of different evidence sources.
In finance, it assesses credit risk or predicts market trends, adjusting estimates based on recent data. Cybersecurity uses it to detect network intrusions by analyzing pattern changes, while in marketing, it personalizes recommendations based on user behavior.
Environmental scientists apply it to improve weather forecasts and monitor ecosystem changes. These applications demonstrate how Bayes’ Theorem provides a logical framework for updating probabilities, enabling smarter decisions across various fields. Additionally, understanding current news can influence decision-making processes in many sectors, emphasizing the importance of staying informed.
Comparing Bayesian and Frequentist Statistical Approaches
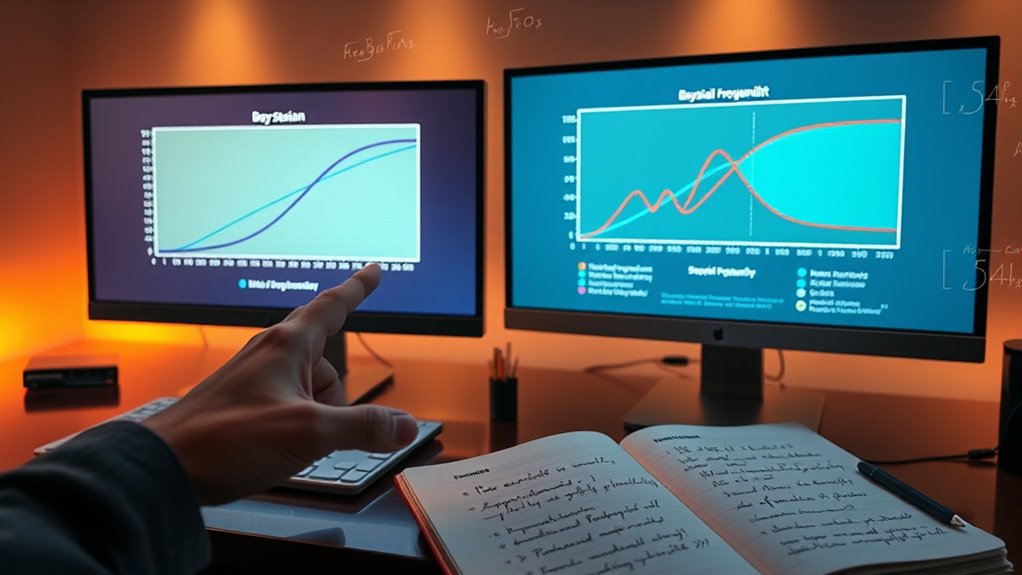
When choosing between Bayesian and frequentist approaches, it’s important to understand how they differ in their interpretation of probability and how they analyze data.
Bayesian statistics updates probabilities with new data, incorporating prior beliefs, and offers a subjective, flexible perspective. Probabilities reflect personal beliefs that change over time. Aura can symbolize the evolving nature of these beliefs, highlighting the dynamic aspect of Bayesian analysis.
Conversely, frequentist methods rely on long-term frequency data, treating probabilities as fixed and objective. They use tools like p-values and null hypothesis testing to evaluate hypotheses, often producing point estimates.
Bayesian outputs include probability distributions, making results more intuitive and interpretable.
In contrast, their seasonal variations can influence the choice of methods, especially in fields like camping or outdoor activities, where environmental factors impact data interpretation.
Frequentist results tend to be binary, focusing on whether data supports a hypothesis.
Your choice depends on data availability, computational resources, and whether you prefer a subjective or objective framework for analysis.
Practical Uses of Bayesian Methods Across Different Fields
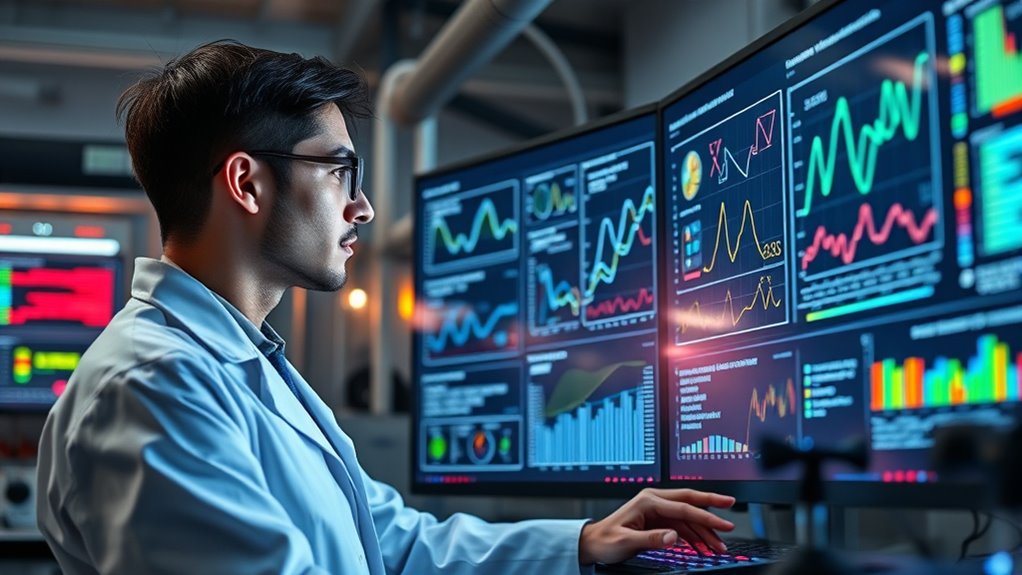
Have you ever wondered how Bayesian methods find practical applications across various fields? In healthcare, they help map disease hotspots, improve diagnoses by combining clinical data with expert knowledge, and create personalized treatments based on individual risks. They also assist in interpreting test results more transparently. Additionally, understanding signs of spoiled lemon juice can prevent health risks associated with spoiled consumables. Bayesian techniques are particularly effective in medical diagnostics, where they update probabilities as new evidence becomes available, leading to more accurate and timely decision-making.
In finance, Bayesian inference predicts market trends, assesses credit risk, and guides portfolio management by updating beliefs with new data. In data science and technology, Bayesian algorithms detect spam, optimize A/B tests, and personalize recommendations. They also enhance natural language processing and machine learning models by integrating prior information.
In environmental sciences, Bayesian models improve weather forecasts, monitor ecosystems, study climate change, and analyze geological data. These versatile methods enable more informed, adaptive decision-making across diverse sectors.
Benefits of Incorporating Prior Knowledge Into Models
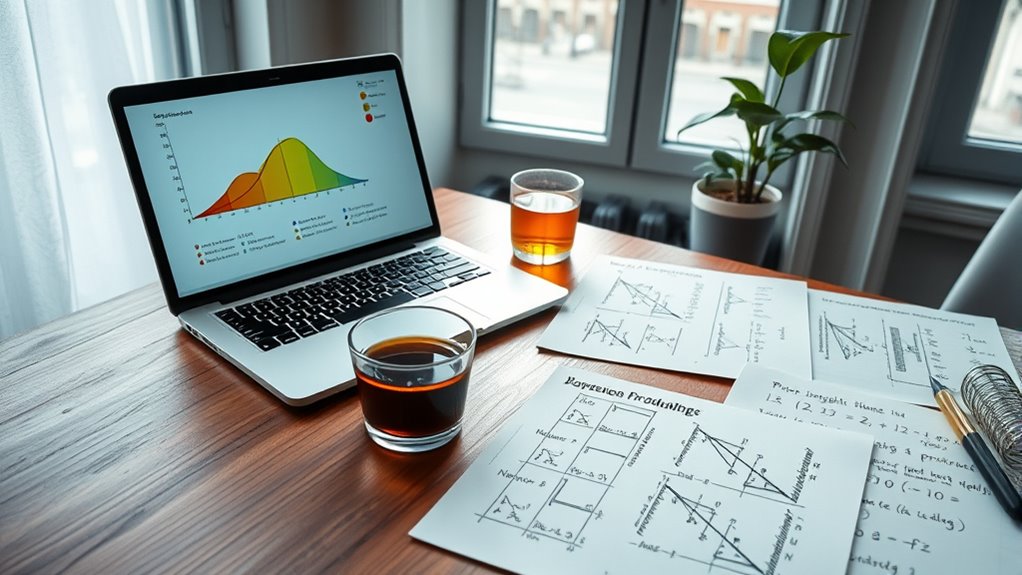
Incorporating prior knowledge into Bayesian models offers significant advantages by leveraging existing information to improve analysis. It enhances parameter estimation, especially when data is sparse, by integrating historical data or expert opinions. Priors help stabilize estimates and prevent unreliable results common with small samples. Comfort solutions for sofa beds can serve as an example of prior knowledge that improves understanding of user preferences and needs. The posterior distribution combines these priors with new data, producing nuanced estimates that reflect both sources. Including prior knowledge also quantifies uncertainty more realistically, which is vital for decision-making under risk. It enables models to learn from past research and adapt over time, supporting sequential updates. Additionally, priors offer flexibility in hypothesis testing and model specification, allowing you to tailor models based on the strength of prior beliefs.
Challenges and Limitations of Bayesian Statistics
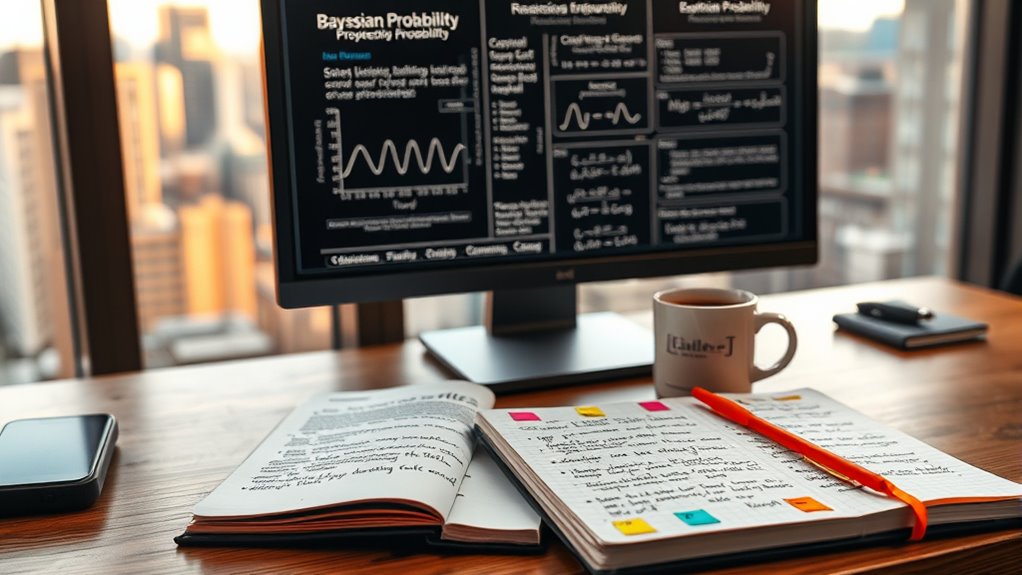
Despite its advantages, Bayesian statistics faces several significant challenges and limitations. You rely heavily on prior knowledge, which can be subjective and influence your results markedly. Choosing appropriate priors isn’t straightforward, and small changes can lead to vastly different outcomes. This sensitivity makes your models less reproducible and more dependent on individual assumptions. Additionally, computational complexity poses a hurdle; methods like Markov Chain Monte Carlo simulations require substantial resources and may not always converge reliably. Techniques such as Markov Chain Monte Carlo algorithms can be computationally intensive and time-consuming. Philosophically, critics question the reliance on priors, arguing it introduces bias and departs from traditional statistical goals. Practically, interpreting Bayesian results can be difficult for non-experts, and integrating these methods into existing frameworks demands expertise and resources. These factors can limit Bayesian approaches in real-world applications. Moreover, the security vulnerabilities associated with complex computational methods can pose risks if not properly managed.
Computational Techniques for Bayesian Data Analysis
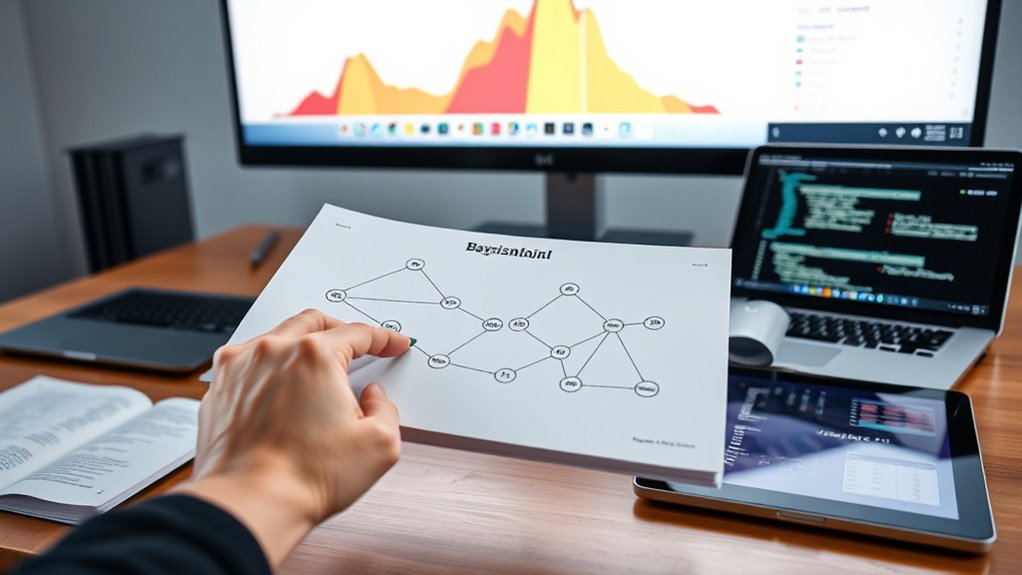
How do you perform Bayesian data analysis when dealing with complex models and intractable integrals? You rely on computational techniques like Markov Chain Monte Carlo (MCMC), which samples from complex posterior distributions when exact solutions are impossible. Monte Carlo methods help approximate difficult integrals, while importance sampling weights samples to improve estimates. Additionally, AI in Business is increasingly integrated into these processes to optimize computation and interpretation. Gibbs sampling, a type of MCMC, simplifies sampling by breaking joint distributions into easier conditional distributions. The Laplace approximation offers a normal approximation around the mode to estimate the posterior efficiently. These methods are complemented by probabilistic programming, which streamlines the implementation of complex Bayesian models. Software tools like RStan, PyStan, JAGS, and PyMC3 facilitate these computations, making Bayesian analysis more accessible. These techniques enable you to handle complex models, perform inference, and visualize results effectively, even when analytical solutions are out of reach.
Future Directions and Innovations in Bayesian Methodologies
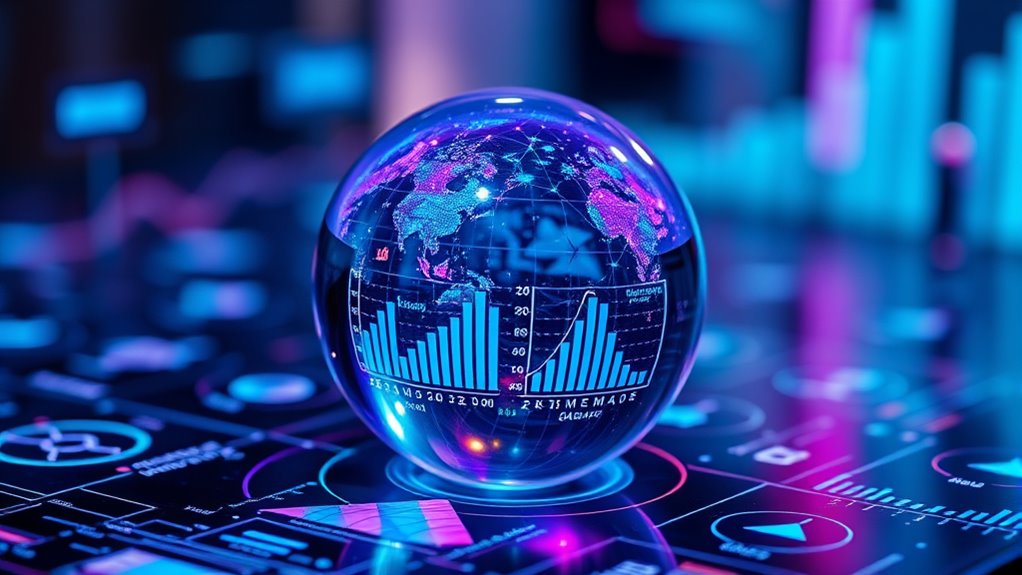
Future directions in Bayesian methodologies are poised to transform how you apply probabilistic reasoning across various fields. In AI and machine learning, you’ll see real-time adaptive systems improve personalized medicine, autonomous vehicles, and smart grids through Bayesian updates. These innovations will also enhance AI content clusters, enabling more precise and interconnected data modeling. Additionally, progress in uncertainty quantification will allow for better decision-making under incomplete or ambiguous information, further broadening Bayesian applications.
Hybrid models combining Bayesian techniques with other paradigms will enable processing multimodal data for deeper insights. Advances in interpretability will help you develop models that balance performance with clarity, addressing ethical concerns.
Quantum Bayesian inference may boost speed and efficiency, while integrating Bayesian methods with deep learning will enhance uncertainty estimation and adaptability. Additionally, innovations in Bayesian meta-analysis will improve decision-making across healthcare, finance, and environmental science by synthesizing diverse data sources and incorporating prior knowledge.
These developments will [UNLOCK] new possibilities for robust, efficient, and transparent probabilistic modeling.
Frequently Asked Questions
How Do I Choose Appropriate Prior Distributions for My Analysis?
When choosing prior distributions, you should consider how much prior knowledge you have. If you’re uncertain, go with non-informative priors like uniform distributions.
If you have reliable data or expert opinions, use informative priors that reflect that knowledge.
Also, think about computational ease and interpretability.
Always perform sensitivity analysis to see how different priors influence your results, ensuring your conclusions are robust.
What Are Common Computational Tools Used in Bayesian Statistics?
When you’re curious about common computational tools in Bayesian statistics, you’ll find a wealth of options. Software like Stan, JAGS, and BUGS excel at sampling and simplifying complex models.
PyMC3 and PyMC4 bring user-friendly interfaces for inference. These tools tackle tackling high-dimensional, hierarchical, and constrained models, making your modeling more manageable.
They optimize accuracy, accelerate analysis, and make Bayesian computation more accessible and adaptable for diverse data and demands.
How Does Bayesian Analysis Handle Small or Limited Datasets?
You find that Bayesian analysis handles small datasets effectively by incorporating prior knowledge through informative priors, which enhances estimate accuracy and reduces bias.
It doesn’t rely on large samples or the central limit theorem, making it more flexible.
You can confidently perform complex models and obtain reliable results even with limited data, thanks to Bayesian methods’ robustness, improved power, and ability to update beliefs as new data becomes available.
Can Bayesian Methods Be Integrated With Machine Learning Algorithms?
You’re asking if Bayesian methods can mesh with machine learning algorithms. The answer is a resounding yes.
You can integrate Bayesian principles into ML models to handle uncertainty, incorporate prior knowledge, and adapt as new data comes in. This fusion makes models more robust, especially when data is scarce or noisy.
What Are the Best Practices for Validating Bayesian Models?
You should use several best practices to validate your Bayesian models. Conduct posterior predictive checks by simulating data from the model and comparing it to observed data.
Perform convergence diagnostics like (hat{R}) and traceplots to guarantee your chains mix well.
Use cross-validation to evaluate performance on new data, and compare moments to check assumptions.
Testing robustness with different priors also helps confirm your model’s reliability.
Conclusion
As you explore Bayesian statistics, imagine your beliefs evolving like a dynamic map, constantly updated with new data. With each piece of evidence, your understanding shifts, revealing hidden patterns and insights. But the journey doesn’t end here. What if future innovations unveil even deeper clarity? The world of Bayesian analysis is full of promise — a fascinating frontier waiting for you to uncover its secrets. Are you ready to take the next step?